Nutritional Assessment: Introduction
1.0 New developments in nutritional assessment
Today, nutritional assessment emphasizes new simple noninvasive approaches, particularly valuable in low income countries, that can measure the risk of nutrient deficits and excesses, and monitor and evaluate the effects of nutrition interventions. These new approaches to assessment include the measurement of nutrients and biomarkers in dried blood spots prepared from a finger-prick blood sample, avoiding the necessity for venous blood collection and refrigerated storage (Mei et al., 2001). In addition, for some nutrients, on-site analysis is now possible, enabling researchers and respondents to obtain results immediately. Many of these new approaches can also be applied to biomarkers monitoring the risk of chronic diseases. These include biomarkers of antioxidant protection, soft-tissue oxidation, and free-radical formation, all of which have numerous clinical applications. Increasingly, “all-in-one” instrumental platforms for multiple micronutrient tests on a single sample aliquot are being developed, some of which have been adapted for dried blood spot matrices (Brindle et al., 2019). These assessment instruments are designed so they are of low complexity and can be operated by laboratory technicians with minimal training, making them especially useful in low- and middle-income countries (Esmaeili et al., 2019). The public availability of e‑ and m‑Health communication technologies has increased dramatically in recent years. e‑Health is defined as:“the use of emerging information and communications technology, especially the internet, to improve or enable health and health care”whereas m-Health interventions are
“those designed for delivery through mobile phones” (Olson, 2016).Interventions using these communication technologies to assess, monitor and improve nutrition-related behaviors and body weight, appear to be efficacious across cognitive outcomes, and some behavioral and emotional outcomes, although changing dietary behaviors is a more challenging outcome. There is an urgent need for a rigorous scientific evaluation of e‑ and m‑health intervention technologies. To date their public health impact remains uncertain. Nutritional assessment is also an essential component of the nutritional care of the hospitalized patient. The important relationship between nutritional status and health, and particularly the critical role of nutrition in recovery from acute illness or injury, is well documented. Although it is many years since the prevalence of malnutrition among hospitalized patients was first reported (Bistrian et al., 1974, 1976), such malnutrition still persists (Barker et al., 2011). In the early 1990s, evidence-based medicine started as a movement in an effort to optimize clinical care. Originally, evidence-based-medicine focused on critical appraisal, followed by the development of methods and techniques for generating systematic reviews and clinical practice guidelines (Djulbegovic and Guyatt, 2017). For more details see Section 1.1.6. Point of care technology (POCT) is also a rapidly expanding health care approach that can be used in diverse settings, particularly those with limited health services or laboratory infrastructure, as the tests do not require specialized equipment and are simple to use. The tests are also quick, enabling prompt clinical decisions to be made to improve the patient’s health at or near the site of patient care. The development and evaluation of POC devices for the diagnosis of malaria, tuberculosis, HIV, and other infectious diseases is on-going and holds promise for low-resource settings (Heidt et al., 2020; Mitra and Sharma, 2021). Guidelines by WHO (2019) for the development of POC devices globally are available, but challenges with regulatory approval, quality assurance programs, and product service and support remain (Drain et al., 2014). Personalized nutrition is also a rapidly expanding approach that tailors dietary recommendation to the specific biological requirements of an individual on the basis of their health status and performance goals. See Setion 1.1.5 for more details. The approach has become possible with the increasing advances in “‑omic sciences” (e.g., nutrigenomics, proteomics and metabolomics). See Chapter 15 and van Ommen et al. (2017) for more details. Health-care administrators and the community in general, continue to demand demonstrable benefits from the investment of public funds in nutrition intervention programs. This requires improved techniques in nutritional assessment and the monitoring and evaluation of nutrition interventions. In addition, implementation research is now being recognized as critical for maximizing the benefits of evidence-based interventions. Implementation research in nutrition aims to build evidence-based knowledge and sound theory to design and implement programs that will deliver nutrition programs effectively. However, to overcome the unique challenges faced during the implementation of nutrition and health interventions, strengthening the capacity of practitioners alongside that of health researchers is essential. Dako-Gyke et al. (2020) have developed an implementation research course curriculum that targets both practitioners and researchers simultaneously, and which is focused on low‑ and middle-income countries. The aim of this 3rd edition of “Principles of Nutritional assessment” is to provide guidance on some of these new, improved techniques, as well as a comprehensive and critical appraisal of many of the classic, well-established methods in nutritional assessment.
1.1 Nutritional assessment systems
Nutritional assessment systems involve the interpretation of information from dietary and nutritional biomarkers, and anthropometric and clinical studies. The information is used to determine the nutritional status of individuals or population groups as influenced by the intake and utilization of dietary substances and nutrients required to support growth, repair, and maintenance of the body as a whole or in any of its parts (Raiten and Combs, 2015). Nutritional assessment systems can take one of four forms: surveys, surveillance, screening, or interventions. These are described briefly below.1.1.1 Nutrition surveys
The nutritional status of a selected population group is often assessed by means of a cross-sectional survey. The survey may either establish baseline nutritional data or ascertain the overall nutritional status of the population. Cross-sectional nutrition surveys can be used to examine associations, and to identify and describe population subgroups “at risk” for chronic malnutrition. Causal relationships cannot be established from cross-sectional surveys because whether the exposure precedes or follows the effect is unknown. They are also unlikely to identify acute malnutrition because all the measurements are taken on a single occasion or within a short time period with no follow-up. Nevertheless, information on prevalence, defined as the proportion who have a condition or disease at one time point, can be obtained from cross-sectional surveys for use by health planners. Cross-sectional surveys are also a necessary and frequent first step in subsequent investigations into the causes of malnutrition or disease. National cross-sectional nutrition surveys generate valuable information on the prevalence of existing health and nutritional problems in a country that can be used both to allocate resources to those population subgroups in need, and to formulate policies to improve the overall nutrition of the population. They are also sometimes used to evaluate nutrition interventions by collecting baseline data before, and at the end of a nutrition intervention program, even though such a design is weak as the change may be attributable to some other factor (Section 1.1.4). Several large-scale national nutrition surveys have been conducted in industrialized countries during the last decade. They include surveys in the United States, the United Kingdom, Ireland, New Zealand, and Australia. More than 400 Demographic and Health Surveys (DHS) in over 90 low‑ and middle-income countries have also been completed. See U.S. DHS program.1.1.2 Nutrition surveillance
The characteristic feature of surveillance is the continuous monitoring of the nutritional status of selected population groups. Surveillance studies therefore differ from nutrition surveys because the data are collected, analyzed, and utilized over an extended period of time. Sometimes, the surveillance only involves specific at‑risk subgroups, identified in earlier nutrition surveys. The information collected from nutrition surveillance programs can be used to achieve the objectives shown in Box 1.1.
Box 1.1 Objectives of nutrition surveillance
Surveillance studies, unlike cross-sectional nutrition
surveys, can also identify the possible causes of both chronic and acute
malnutrition and, hence, can be used to
formulate and initiate intervention measures
at either the population or the subpopulation level.
In the United States, a comprehensive program of national
nutrition surveillance, known as the
National Health and Nutrition Examination Survey
(NHANES),
has been conducted since 1959.
Data on anthropometry, demographic and socio-economic status, dietary and
health-related measures are collected. In 2008, the United Kingdom began the
National Diet and Nutrition Survey Rolling Program.
This is a continuous program of field work designed to assess the diet,
nutrient intake, and nutritional status of the general population aged 1.5y
and over living in private households in the U.K.
(Whitton et al., 2011).
WHO has provided some countries with
surveillance systems
so that they can monitor changes in the global targets to reduce the high
burden of disease associated with malnutrition.
Note that the term “nutrition monitoring,”
rather than nutrition surveillance, is often
used when the participants selected are high‑risk
individuals (e.g., food‑insecure households, pregnant women).
For example, because household food insecurity is of increasing public health
concern, even in high-income countries
such as the U.S. and Canada, food insecurity is regularly
monitored in these countries using the Household Food Security Survey Module
(HFSSM).
Also see: Loopstra
(2018).
- Aid long-term planning in health and development;
- Provide input for program management and evaluation;
- Give timely warning of the need for intervention to prevent critical deteriorations in food consumption.
- population-based;
- decision and action orientated;
- sensitive and accurate;
- relevant and timely;
- readily accessible;
- communicated effectively.
1.1.3 Nutrition screening
The identification of malnourished individuals requiring intervention can be accomplished by nutrition screening. This involves a comparison of measurements on individuals with predetermined risk levels or “cutoff” points using measurements that are accurate, simple and cheap (Section 1.5.3), and which can be applied rapidly on a large scale. Nutrition screening can be carried out on the whole population, targeted to a specific subpopulation considered to be at risk, or on selected individuals. The programs are usually less comprehensive than surveys or surveillance studies. Numerous nutrition screening tools are available for the early identification and treatment of malnutrition in hospital patients and nursing homes, of which Subjective Global assessment (SGA) and the Malnutrition Universal Screening Tool (MUST) are widely used; see Barker et al. (2011) and Chapter 27 for more details. In low-income countries, mid‑upper‑arm circumference (MUAC) with a fixed cutoff of 115mm is often used as screening tool to diagnose severe acute malnutrition (SAM) in children aged 6–60mos (WHO/UNICEF, 2009). In some settings, mothers have been supplied with MUAC tapes either labeled with a specific cutoff of < 115mm, or color-coded in red (MUAC < 115mm), yellow (MUAC = 115–124mm), and green (MUAC > 125mm) in an effort to detect malnutrition early, before the onset of complications, and thus reduce the need for inpatient treatment (Blackwell et al., 2015; Isanaka et al., 2020). In the United States, screening is used to identify individuals who might benefit from the Supplemental Nutrition Assistance Program (SNAP). The program is means tested with highly selective qualifying criteria. The SNAP (formerly food stamps) program provides money loaded onto a payment card which can be used to purchase eligible foods, to ensure that eligible households do not go without foods. In general, studies have reported that participation in SNAP is associated with a significant decline in food insecurity (Mabli and Ohls, 2015). The U.S. also has a Special Supplemental Nutrition Program for Women, Infants, and Children (WIC) that targets low-income pregnant and post-partum women, infants, and children < 5y. In 2009, the USDA updated the WIC food packages in an effort to balance nutrient adequacy with reducing the risk of obesity; details of the updates are available in NASEM (2006). Guthrie et al. (2020) compared associations between WIC participants and the nutrients and food packages consumed in 2008 and in 2016 using data from cross-sectional nationwide surveys of children aged < 4y. The findings indicated that more WIC infants who received the updated WIC food packages in 2016 had nutrient intakes (except iron) that met their estimated average requirements (EARs). Moreover, vegetables provided a larger contribution to their nutrient intakes, and intakes of low‑fat milks had increased for toddlers aged 2y, likely contributing to their lower reported intakes of saturated fat.1.1.4 Nutrition interventions
Nutrition interventions often target population subgroups identified as “at‑risk” during nutrition surveys or by nutrition screening. In 2013, the Lancet Maternal and Child Nutrition Series recommended a package of nutrition interventions that, if scaled to 90% coverage, could reduce stunting by 20% and reduce infant and child mortality by 15% (Bhutta et al., 2013). The nutrition interventions considered included lipid-based and micronutrient supplementation, food fortification, promotion of exclusive breast feeding, dietary approaches, complementary feeding, and nutrition education. More recently, nutrition interventions that address nutrition-sensitive agriculture are also being extensively investigated (Sharma et al., 2021) Increasingly, health-care program administrators and funding agencies are requesting evidence that intervention programs are implemented as planned, reach their target group in a cost-effective manner, and are having the desired impact. Hence, monitoring and evaluation are becoming an essential component of all nutrition intervention programs. However, because the etiology of malnutrition is multi-factorial and requires a multi-sectorial response, the measurement and collection of the data from such multiple levels presents major challenges. Several publications are available on the design, monitoring, and evaluation of nutrition interventions. The reader is advised to consult these sources for further details (Habicht et al., 1999; Rossi et al., 1999; Altman et al., 2001). Only a brief summary is given below. Monitoring, discussed in detail by Levinson et al. (1999), oversees the implementation of an intervention, and can be used to assess service provision, utilization, coverage, and sometimes the cost of the program. Effective monitoring is essential to demonstrate that any observed result is probably from the intervention. Emphasis on the importance of designing a program theory framework and associated program impact pathway (PIP) to understand and improve program delivery, utilization, and the potential of the program for nutritional impact has increased (Olney et al., 2013; Habicht and Pelto, 2019). The construction of a PIP helps conceptualize the program and its different components (i.e., inputs, processes, outputs, and outcomes to impacts). Only with this information can issues in program design, implementation, or utilization that may have the potential to limit the impact of the program, be identified, and, in turn strengthened, so the impact of the program can be optimized. Program impact pathway analysis generally includes both quantitative and qualitative methods (e.g., behavior-change communication) to ascertain the coverage of an intervention. An example of the multiple levels of measurements and data that were collected to optimize the impact of a “Homestead Food Production” program conducted in Cambodia are itemized in Box 1.2. Three program impact pathways were hypothesized, each requiring the measurements of a set of input, process, and output indicators; for more details of the indicators measured, see Olney et al. (2013).
Box 1.2 Example of the three hypothesized program impact pathways
Program impact pathway analysis can also be used to ascertain the coverage
of an intervention. Bottlenecks at each sequential step along the PIP can be
identified along with the potential determinants of the bottlenecks
(
Habicht and Pelto, 2019).
Coverage can be measured at the individual and at the population
level; in the latter case, it is assessed as the proportion
of beneficiaries who received the
intervention at the specified quality level.
Many of the nutrition interventions highlighted by Bhutta and colleagues
(2013)
in the Lancet Maternal and Child Nutrition Series have now been
incorporated into national policies and programs in
low‑ and middle-income countries. However, reliable data on their coverage are
scarce, despite the importance of coverage to ensure
sustained progress in reducing rates of
malnutrition. In an effort to achieve this goal, Gillespie et al.
(2019)
have proposed a set of indicators for tracking
the coverage of high-impact nutrition-specific
interventions which are delivered primarily through health systems, and
recommend incorporation of these indicators
into data collection mechanisms and
relevant intervention delivery platforms.
For more details, see Gillespie et al.
(2019).
The evaluation of any nutrition intervention program requires the choice of an
appropriate design to assess the
performance or effect of the intervention. The
choice of the design depends on the purpose of the evaluation
and the level of precision required.
For example, for large scale public health
programs, based on the evaluation, decisions may be made to continue, expand,
modify, strengthen, or discontinue the program; these
aspects are discussed in detail by Habicht et al.
(1999).
The indicators used to address
the evaluation objectives must also be carefully considered
(Habicht & Pelletier, 1990;
Habicht & Stoltzfus, 1997).
Designs used for nutrition
interventions vary in their complexity; see Hulley et al.
(2013)
for more details. Three types of evaluation can be achieved from these
designs: adequacy, plausibility and probability evaluation, each of which is
addressed briefly below.
An adequacy evaluation is achieved
when it has not been feasible to include a
comparison or control group in the intervention design.
Instead, a within-group design has been used. In these circumstances,
the intervention is evaluated on the basis of whether the expected changes
have occurred by comparing the outcome in
the target group with either a previously defined
goal, or with the change observed in the
target group following the intervention
program. An example might be
distributing iron supplements to all the target
group (e.g., all preschool children with iron deficiency
anemia) and assessing whether the goal
of < 10% prevalence of iron-deficiency
anemia in the intervention area after two
years, has been met. Obviously, when evaluating
the outcome by assessing the adequacy
of change over time, at least baseline and final
measurements are needed. Note that because
there is no control group in this design, any
reported improvement in the group, even if it
is statistically significant, cannot be causally
linked to the intervention.
A plausibility evaluation
can be conducted with several designs, including a
nonrandomized between-group design, termed a quasi-experimental design in
which the experimental group receives the intervention, but the
control group does not. The design should
preferably allow blinding (e.g., use an identical
placebo). Because the participants are not randomized
into the two groups, multivariate
analysis is used to control for potential confounding
factors and biases, although it may
not be possible to fully remove these statistically.
A between-group quasi-experimental
design requires more resources and is therefore
more expensive than the within-group
design discussed earlier, and is used when decision
makers require a greater degree of confidence
that the observed changes are indeed due to the intervention program.
A probability evaluation,
when properly executed, provides the highest level of
evidence that the intervention caused the outcome, and is considered the gold
standard method. The method requires the use of a randomized,
controlled, double-blind experimental design, in which
the participants are randomly assigned
to either the intervention or the control
group. Randomization is conducted to ensure
that, within the limits of chance, the treatment
and control groups will be comparable at
the start of the study. In some randomized trials,
the treatment groups are communities and not
individuals, in which case they are known as
“community” trials.
Figure 1.1
illustrates the importance of the participants being randomized to
either the intervention or the control group
when the control group outcomes have also
improved as a result of nonprogram factors.
- Pathway 1: Increasing the availability of micronutrient-rich foods through increased household production of these foods (production-consumption pathway)
- Pathway 2: Income generation through the sale of products from the homestead food production program (production–income pathway)
- Pathway 3: Increased knowledge and adoption of optimal nutrition practices, including intake of micronutrient-rich foods (knowledge–adoption of optimal health- and nutrition-related practices pathway) and improve delivery, utilization, and potential for impact of a Homestead Food Production Program in Cambodia.
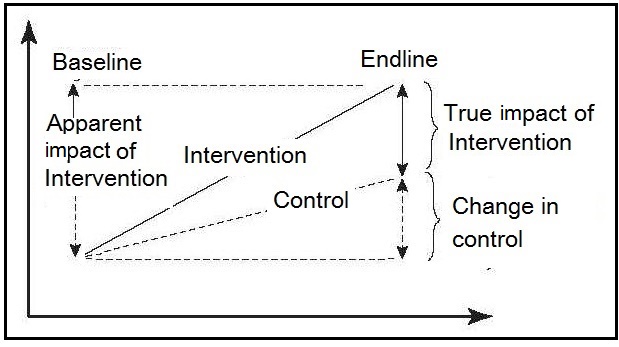
1.1.5 assessment systems in a clinical setting
The types of nutritional assessment systems used in the community have been adopted in clinical medicine to assess the nutritional status of hospitalized patients. This practice has arisen because of reports of the high prevalence of protein-energy malnutrition among surgical patients in North America and elsewhere (Corish and Kennedy, 2000; Barker et al., 2011). Today, nutritional assessment is often performed on patients with acute traumatic injury, on those undergoing surgery, on chronically ill medical patients, and on elderly patients. Initially, screening can be carried out to identify those patients requiring nutritional management. A more detailed and comprehensive baseline nutritional assessment of the individual may then follow. This assessment will clarify and expand the nutritional diagnosis, and establish the severity of the malnutrition. Finally, a nutrition intervention may be implemented, often incorporating nutritional monitoring and an evaluation system, to follow both the response of the patient to the nutritional therapy and its impact. Further details of protocols that have been developed to assess the nutritional status of hospital patients are given in Chapter 27. Personalized nutrition is also a rapidly expanding approach that is being used in a clinical setting, as noted earlier. The approach tailors dietary recommendation to the specific biological requirements of an individual on the basis of their health status and performance goals. The latter are not restricted to the prevention and/or mitigation of chronic disease but often extend to strategies to achieve optimal health and well-being; some examples of these personal goals are depicted in Table 1.1.Goal | Definition |
---|---|
Weight management | Maintaining (or attaining) an ideal body weight and/or body shaping that ties into heart, muscle, brain and metabolic health |
Metabolic health | Keeping metabolism healthy today and tomorrow |
Cholesterol | Reducing and optimizing the balance between
high-density lipoprotein and low-density lipoprotein cholesterol in individuals in whom this is disturbed |
Blood pressure | Reducing blood pressure in individuals who have
elevated blood pressure |
Heart health | Keeping the heart healthy today and tomorrow. |
Muscle | Having muscle mass and muscle functional abilities. This is the physiological basis or underpinning of the consumer goal of “strength” |
Endurance | Sustaining energy to meet the challenges of the day (e.g., energy to do that report at work, energy to play soccer with your children after work) |
Strength | Feeling strong within yourself,
avoiding muscle fatigue |
Memory | Maintaining and attaining an optimal short-term and/or working memory |
Attention | Maintaining and attaining optimal focused and
sustained attention (i.e., being “in the moment” and able to utilize information from that “moment”) |
1.1.6 Approaches to evaluate the evidence from nutritional assessment studies
In an effort to optimize clinical care, evidence-based medicine (EBM) started as a movement in the early 1990s to enhance clinician's understanding, critical thinking, and use of the published research literature, while at the same time considering the patient’s values and preferences. It focused on the quality of evidence and risk of bias associated with the types of scientific studies used in nutritional assessment as shown in the EBM hierachy of evidence pyramid in Figure 1.2, with randomized controlled trials (RCTs) providing the strongest evidence and hence occupying the top tier.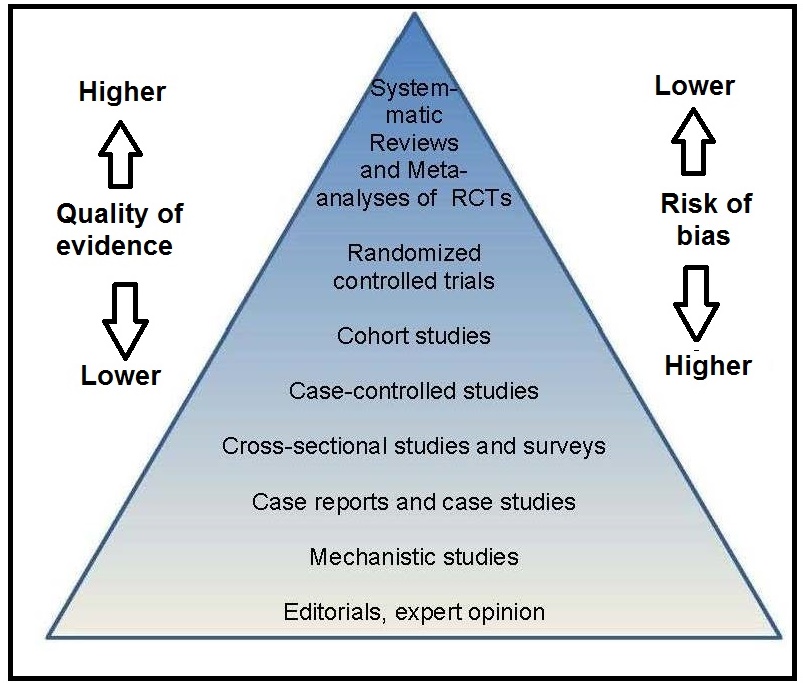
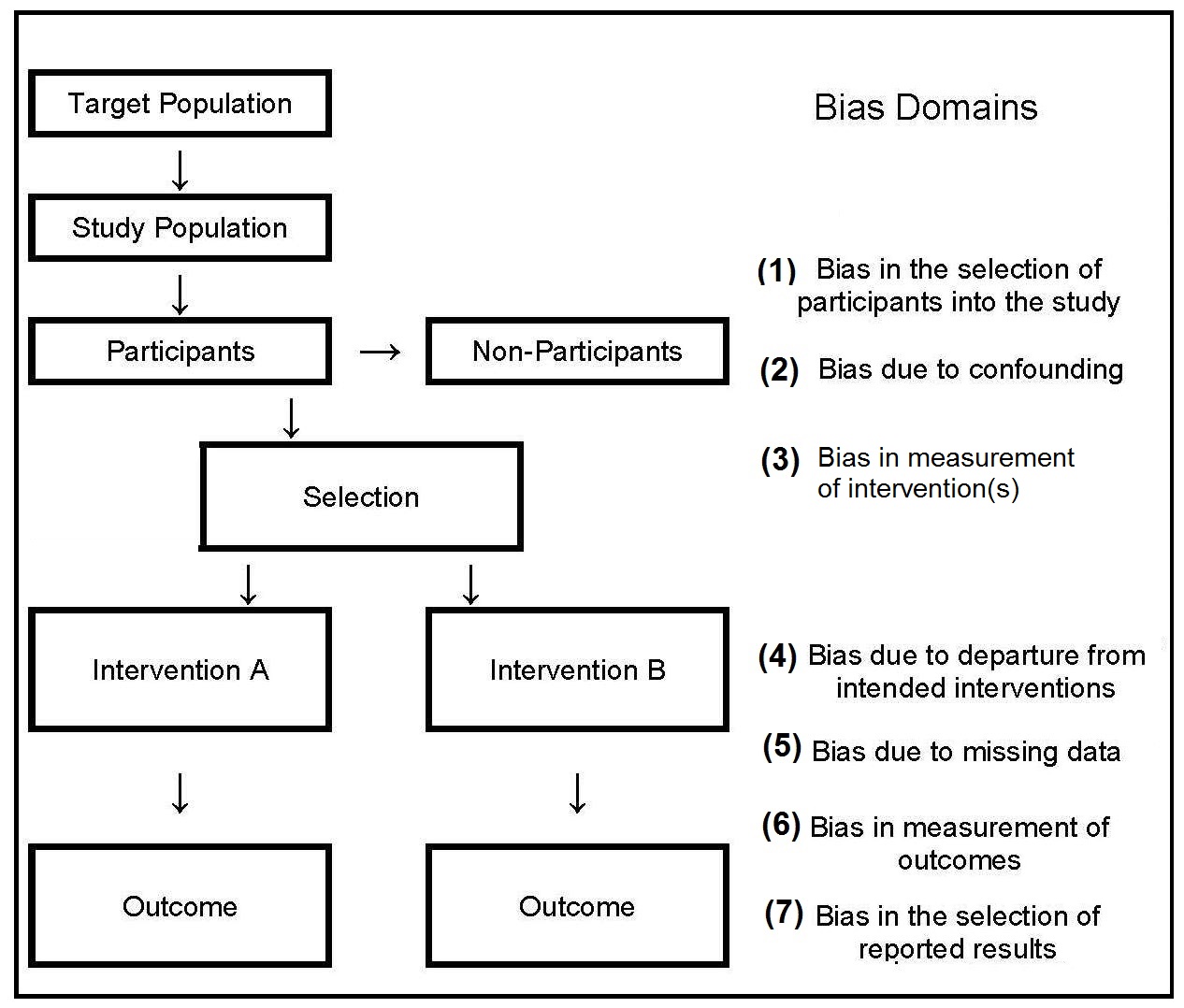
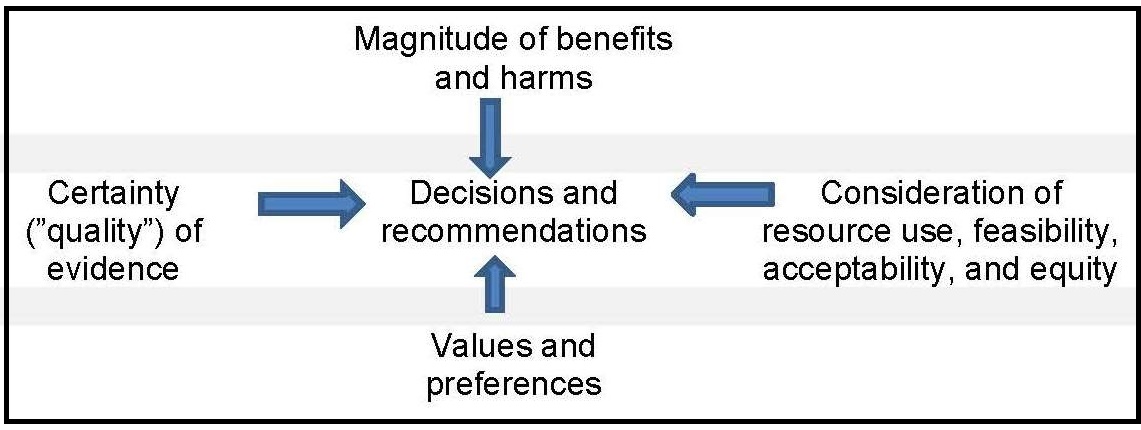
Box 1.3 Steps in a systematic review
Systematic reviews that address nutrition questions
present some unique challenges. Approaches that can
be used to address some of these challenges are
summarized in
Table 1.2
- Prepare the topic — refine questions and develop an analytic framework
- Search for and select studies — identify eligibility criteria, search for relevant studies, and select evidence for inclusion
- Abstract data — extract evidence from studies and construct evidence tables
- Analyze and synthesize data — critically assess quality of studies using a prespecified method, assess applicability of studies, apply qualitative methods, apply quantitative methods such as meta-analysis, and rate the strength of a body of evidence
- Present findings
Challenge | Approach |
---|---|
Baseline exposure | Unlike drug exposure,
most persons have some level of dietary exposure to the nutrient or dietary substance of interest, either from food or supplements, or by endogenous synthesis in the case of vitamin D, information on background intakes and the methodologies used to assess them should be captured in the SR so that any related uncertainties can be factored into data interpretation. |
Nutrient status | The nutrient status of an individual or population can affect the response to nutrient supplementation. |
Chemical
form of the nutrient or dietary substance | If nutrients occur in multiple forms,
the forms may differ in their biological activity. Assuring bioequivalence or making use of conversion factors can be critical for appropriate data interpretation. |
Factors that influence bioavailability | Depending upon the
nutrient or dietary substance, influences such as nutrient-nutrient interactions, drug or food interactions, adiposity, or physiological state such as pregnancy may affect the utilization of the nutrient. Capturing such information allows these influences to be factored into conclusions about the data. |
Multiple and interrelated biological functions of a nutrient or dietary substance | Biological functions need to be
understood in order to ensure focus and to define clearly the nutrient- or dietary substance—specific scope of the review. |
Nature of nutrient or dietary substance intervention | Food-based interventions require detailed documentation of the approaches taken to assess nutrient or dietary substance intake. |
Uncertainties in assessing dose- response relationships | Specific documentation of measurement and assay procedures is required to account for differences in health outcomes. |
1.2 Nutritional assessment methods
Historically, nutritional assessment systems have focused on methods to characterize each stage in the development of a nutritional deficiency state. The methods were based on a series of dietary, laboratory-based biomarkers, anthropometric, and clinical observations used either alone or, more effectively, in combination. Today, these same methods are used in nutritional assessment systems for a wide range of clinical and public health applications. For example, many low and middle-income countries are now impacted by a triple burden of malnutrition, where undernutrition, multiple micronutrient deficiencies, and overnutrition co-exist. Hence, nutritional assessment systems are now applied to define multiple levels of nutrient status and not just the level associated with a nutrient deficiency state. Such levels may be associated with the maintenance of health, or with reduction in the risk of chronic disease; sometimes, levels leading to specific health hazards or toxic effects are also defined (Combs, 1996). There is now increasing emphasis on the use of new functional tests to determine these multiple levels of nutrient status. Examples include functional tests that measure immune function, muscle strength, glucose metabolism, nerve function, work capacity, oxidative stress, and genomic stability (Lukaski and Penland, 1996; Mayne, 2003; Russell, 2015; Fenech, 2003). The correct interpretation of the results of nutritional assessment methods requires consideration of other factors in addition to diet and nutrition. These may often include socioeconomic status, cultural practices, and health and vital statistics, which collectively are sometimes termed “ecological factors”; see Section 1.2.5. When assessing the risk of acquiring a chronic disease, environmental and genetic factors are also important (Yetley et al., 2017a).1.2.1 Dietary methods
Dietary assessment methods provide data used to describe exposure to food and nutrient intakes as well as information on food behaviors and eating patterns that cannot be obtained by any other method. The data obtained have multiple uses for supporting health and preventing disease. For example, health professionals use dietary data for dietary counseling and education and for designing healthy diets for hospitals, schools, long-term care facilities and prisons. At the population level, national food consumption surveys can generate information on nutrient adequacy within a country, identify population groups at risk, and develop nutrition intervention programs. Dietary data can also be used by researchers to study relationships between diet and disease, and for formulating nutrition policy such as food-based dietary guidelines (Murphy et al., 2016). It is important to recognize that nutrient inadequacies may arise from a primary deficiency (low levels in the diet) or because of a secondary deficiency. In the latter case, dietary intakes may appear to meet nutritional needs, but conditioning factors (such as certain drugs, dietary components, or disease states) interfere with the ingestion, absorption, transport, utilization, or excretion of the nutrient(s). Several dietary methods are available, the choice depending primarily on both the study objectives and the characteristics of the study group (see Chapter 3 for more details). Recently, many technical improvements have been developed to improve the accuracy of dietary methods. These include the use of digital photographs of food portions displayed on a cell-phone or a computer tablet, or image-based methods utilizing video cameras, some wearable. Some of these methods rely on active image capture by users, and others on passive image capture whereby pictures are taken automatically. Under development are wearable camera devices which objectively measure diet without relying on user-reported food intake (Boushy et al., 2017). Several on-line dietary assessment tools are also available, all of which standardize interview protocols and data entry: they can be interviewer‑ or self‑administered (Cade, 2017); see Chapter 3 for more details. Readers are advised to consult Intake — a Center for Dietary assessment that provides technical assistance for the planning, collection, analysis and use of dietary data. Examples of their available publications are presented in Box 1.4. In addition, recommendations for collecting, analyzing, and interpreting dietary data to inform dietary guidance and public health policy are also available; see Murphy et al., (2016) and Subar et al., (2015) for more details.
Box 1.4 Examples of publications by Intake.org
Data on knowledge, attitudes and practices,
and reported food‑related behaviors are
also collected. Historically, this has involved observing the participants,
as well as in‑depth interviews and
focus groups — approaches based on ethnological
and anthropological techniques. Today, e‑health (based on the internet)
and m‑health (based on mobile phones) communication technologies are
also being used to collect these data, as noted earlier
(Olson, 2016).
All these methods are particularly useful
when designing and evaluating nutrition interventions.
Often, information on the proportion of the population “at
risk” of inadequate intakes of nutrients is required.
Such information can be used to ascertain whether assessment
using more invasive methods based on nutritional
biomarkers are warranted in a specific population or subgroup.
- Considerations for the selection of Portion Size Estimation Methods for Use in Quantitative 24-Hour Dietary Recall Surveys in low‑ and Middle-Income Countries. (Vossenaar et al. 2020)
- Estimating Usual Intakes from Dietary Surveys: Methodologic Challenges, Analysis Approaches, and Recommendations for low‑ and Middle-Income Countries. (Tooze, 2020)
- Guidance for the Development of Food Photographs for Portion Size Estimation in Quantitative 24-Hour Dietary Recall Surveys in low‑ and Middle-Income Countries. (Vossenaar et al. 2020)
- CSDietary Software Program .CSDietary HarvestPlus, SerPro S.A. (CSDietary, 2020)
- Intake Survey Guidance Document: Estimating Usual Intakes from Dietary Surveys — Methodologic Challenges, Analysis Approaches, and Recommendations for LMICs (Tooze, 2020)
- Dietary Survey Protocol Template: An Outline to Assist with the Development of a Protocol for a Quantitative 24-Hour Dietary Recall Survey in a low‑ or Middle-Income Country. (Dietary Recall, 2020)
- An Overview of the Main Pre-Survey Tasks Required for Large-Scale Quantitative 24-Hour Recall Dietary Surveys in LMICs (Vossenaar et al., 2020)
1.2.2 Laboratory Methods
Laboratory methods are used to measure nutritional biomarkers which are used to describe status, function, risk of disease, and response to treatment. They can also be used to describe exposure to certain foods or nutrients, when they are termed “dietary biomarkers”. Most useful are nutritional biomarkers that distinguish deficiency, adequacy and toxicity, and which assess aspects of physiological function and/or current or future health. However, it must be recognized that a nutritional biomarker may not be equally useful across different applications or life-stage groups where the critical function of the nutrient or the risk of disease may be different (Yetley et al., 2017b). The Biomarkers of Nutrition and Development (BOND) program (Raiten and Combs, 2015) has defined a nutritional biomarker as:“a biological characteristic that can be objectively measured and evaluated as an indicator of normal biological or pathogenic processes, and/or as an indicator of responses to nutrition interventions”.Nutritional biomarkers can be measurements based on biological tissues and fluids, on physiological or behavioral functions and, more recently, on metabolic and genetic data that in turn influence health, well-being, and risk of disease. Yetley and colleagues (2017b) have highlighted the difference between risk biomarkers and surrogate biomarkers. A risk biomarker is defined by the Institute of Medicine (2010) as a biomarker that indicates a component of an individual’s level of risk of developing a disease or level of risk of developing complications of a disease. As an example, metabolomics is being used to investigate potential risk biomarkers of pre-diabetes that are distinct from the known diabetes risk indicators (glycosylated hemoglobin levels, fasting glucose, and insulin) (Wang-Sattler et al., 2012). BOND classified nutritional biomarkers into three groups shown in Box 1.5,
Box 1.5. Classification of
nutritional biomarkers
based on the assumption that an intake-response
relationship exists between the
biomarkers of exposure (i.e., nutrient intake)
and the biomarkers of status and
function. Functional physiological and
behavioral biomarkers are more directly
related to health status and disease than are
the functional biochemical biomarkers
shown in Box 1.5. Disturbances in these functional physiological and behavioral
biomarkers are generally associated with
more prolonged and severe nutrient
deficiency states, and are often affected by
social and environmental factors so their
sensitivity and specificity are low.
In general, functional physiological
tests (with the exception of
physical growth) are not suitable for
large-scale nutrition surveys:
they are often too invasive, they may
require elaborate equipment, and the results
tend to be difficult to interpret
because of the lack of cutoff points. Details of
functional physiological or behavioral tests dependent
on specific nutrients are summarized
in
Chapters 16–25.
The growing prevalence of chronic diseases has led to
investigations to identify biomarkers that can be used as substitutes for
chronic disease outcomes
(Yetley et al., 2017b).
Chronic disease events are
characterized by long developmental times, and are
multifactorial in nature with challenges in
differentiating between casual and associative relations
(Yetley et al., 2017b).
To qualify as a biomarker that is intended to
substitute for a clinical endpoint, the biomarker must be on the major
causal pathway between an intervention (e.g., diet or dietary component)
and the outcome of interest (e.g., chronic disease). Such biomarkers are
termed “surrogate” biomarkers; only a few such
biomarkers have been identified for
chronic disease. Examples of well accepted
surrogate biomarkers are blood
pressure within the pathway of sodium intake
and cardiovascular disease (CVD) and low
density lipoprotein-cholesterol (LDL)
concentration within a saturated fat and CVD
pathway; see Yetley et al
(2017b)
for more details.
Increasingly, it is recognized that a
single biomarker may not reflect exclusively
the nutritional status of that single
nutrient, but instead be reflective of several
nutrients, their interactions, and metabolism.
This has led to the development of
“all‑in‑one” instrument platforms
that conduct multiple micronutrient tests in a
single sample aliquot, as noted earlier.
A 7‑plex microarray immunoassay has been
developed for ferritin, soluble
transferrin receptor, retinol binding protein,
thyroglobulin, malarial antigenemia and
inflammation status biomarkers
(Brindle et al., 2019),
which has subsequently been
applied to dried blood spot matrices
(Brindle et al., 2019).
Comparisons with reference‑type assays indicate that with
some improvements in accuracy and
precision, these multiplex instrument platforms could
be useful tools for assessing multiple
micronutrient biomarkers in national
micronutrient surveys in low
resource settings
(Esmaeili et al., 2019).
Readers are advised to consult the Micronutrient Survey Manual and Toolkit
developed by the U.S. Centers for Disease Control and Prevention (CDC)
for details on planning, implementation, analysis, reporting, dissemination and the
use of data generated from a national cross-sectional
micronutrient survey. For details, see
(CDC, 2020).
-
Biomarkers of “exposure”: food or nutrient intakes;
dietary patterns; supplement usage. Assessed by:
- Traditional dietary assessment methods
- Dietary biomarkers: indirect measures of nutrient exposure
- Biomarkers of “status”: body fluids (serum, erythrocytes, leucocytes, urine, breast milk); tissues (hair, nails)
-
Biomarkers of “function":
measure the extent of the functional consequences of a
nutrient deficiency.
- Functional biochemical: enzyme stimulation assays; abnormal metabolites; DNA damage. These biomarkers serve as early biomarkers of subclinical deficiencies.
- Functional physiological/behavioral: more directly related to health status or disease such as vision, growth, immune function, taste acuity, cognition, depression. These biomarkers impact on clinical and health outcomes.
Exposure
→
Status
→
Function
→
Outcomes
1.2.3 Anthropometric methods
Anthropometric methods involve measurements of the physical dimensions and gross composition of the body (WHO, 1995). The measurements vary with age (and sometimes with sex and race) and degree of nutrition, and they are particularly useful in circumstances where chronic imbalances of protein and energy are likely to have occurred. Such disturbances modify the patterns of physical growth and the relative proportions of body tissues such as fat, muscle, and total body water. In some cases, anthropometric measurements can detect moderate and severe degrees of malnutrition, but cannot identify specific nutrient deficiency states. The measurements provide information on past nutritional history, which cannot be obtained with equal confidence using other assessment techniques. Anthropometry is used in both clinical and public health settings to identify the increasing burden of both under- and over-nutrition that now co-exist, especially in low‑ and middle-income countries. Measurements can be performed relatively quickly, easily, and reliably using portable equipment, provided standardized methods and calibrated equipment are used (Chapters 10 and 11). To aid in their interpretation, the raw measurements are generally expressed as an index, such as height-for-age (See Section 1.3). Standardized methods exist to evaluate anthropometric indices based on Z‑scores or percentiles, both calculated in relation to the distribution of the corresponding anthropometric index for the healthy reference population (Section 1.5.1 and Section 1.5.2). Often Z‑scores of below −2 or above +2 are used to designate individuals with either unusually low or unusually high anthropometric indices, especially in low income countries. When used in this way, the combination of index and reference limit is termed an “indicator”, a term that relates to their use in nutritional assessment, often for public health, or social/medical decision-making (see Chapter 13 for more details). There is growing concern about the global pandemic of obesity; individuals with obesity are at higher risk of several chronic diseases, including coronary heart disease, diabetes, and hypertension. Consequently, numerous investigators have compared the usefulness of anthropometric variables such as body mass index (weight,kg) / (height, m)2 (BMI) and waist circumference as surrogate measures of obesity. In a meta-analysis of studies with at least a 12mos follow-up, Seo et al. (2017) concluded that waist circumference was a better predictor for diabetes than BMI (> 30) in women than men and for all ages > 60y, whereas neither BMI > 30, nor waist circumference > 102cm (for men), > 88cm (for women) were significant predictors of hypertension.1.2.4 Clinical methods
A medical history and a physical examination are the clinical methods used to detect signs, (observations made by a qualified examiner) and symptoms (manifestations reported by the patient) associated with malnutrition or risk of chronic disease. The latter is defined by IOM (2010) as a culmination of a series of pathogenic processes in response to internal or external stimuli over time that results in a clinical diagnosis/ailment and health outcomes; examples include diabetes, cancer, coronary heart disease, stroke, and arthritis. The signs and symptoms may be nonspecific and develop only during the advanced stages of a nutrient deficiency (or excess) or chronic disease; for this reason, their diagnosis should not rely exclusively on clinical methods. It is obviously desirable to have the capacity to detect marginal nutrient deficiencies and risk of chronic disease before a clinical syndrome develops. Several laboratory-based biomarkers exist to assess an individual’s level of risk of developing a disease and as substitutes for chronic disease outcomes; they are often included as an adjunct to clinical assessment. Examples include serum ferritin for risk of iron deficiency anemia, glycosylated hemoglobin (HbA1c) for risk of diabetes, and alterations in bone mineral density for changes in fracture risk. Examples of surrogate biomarkers intended to substitute for chronic disease outcomes include LDL cholesterol instead of the true clinical outcome CVD and blood pressure for cardiovascular disease, as noted earlier (Yetley et al., 2017b).1.2.5 Ecological factors
Increasingly, nutritional assessment methods include the collection of information on a variety of other factors known to influence the nutritional status of individuals or populations. This increase has stemmed, in part, from the the United Nations Children’s Fund (UNICEF) conceptual framework for the causes of childhood malnutrition shown in Figure 1.5, and the increasing focus on studies of diet and chronic disease (Yetley et al., 2017a). The UNICEF framework highlights that child malnutrition is the outcome of a complex causal process involving not just the immediate determinants such as inadequate dietary intake and poor care, but also the underlying and basic enabling determinants depicted in Figure 1.5.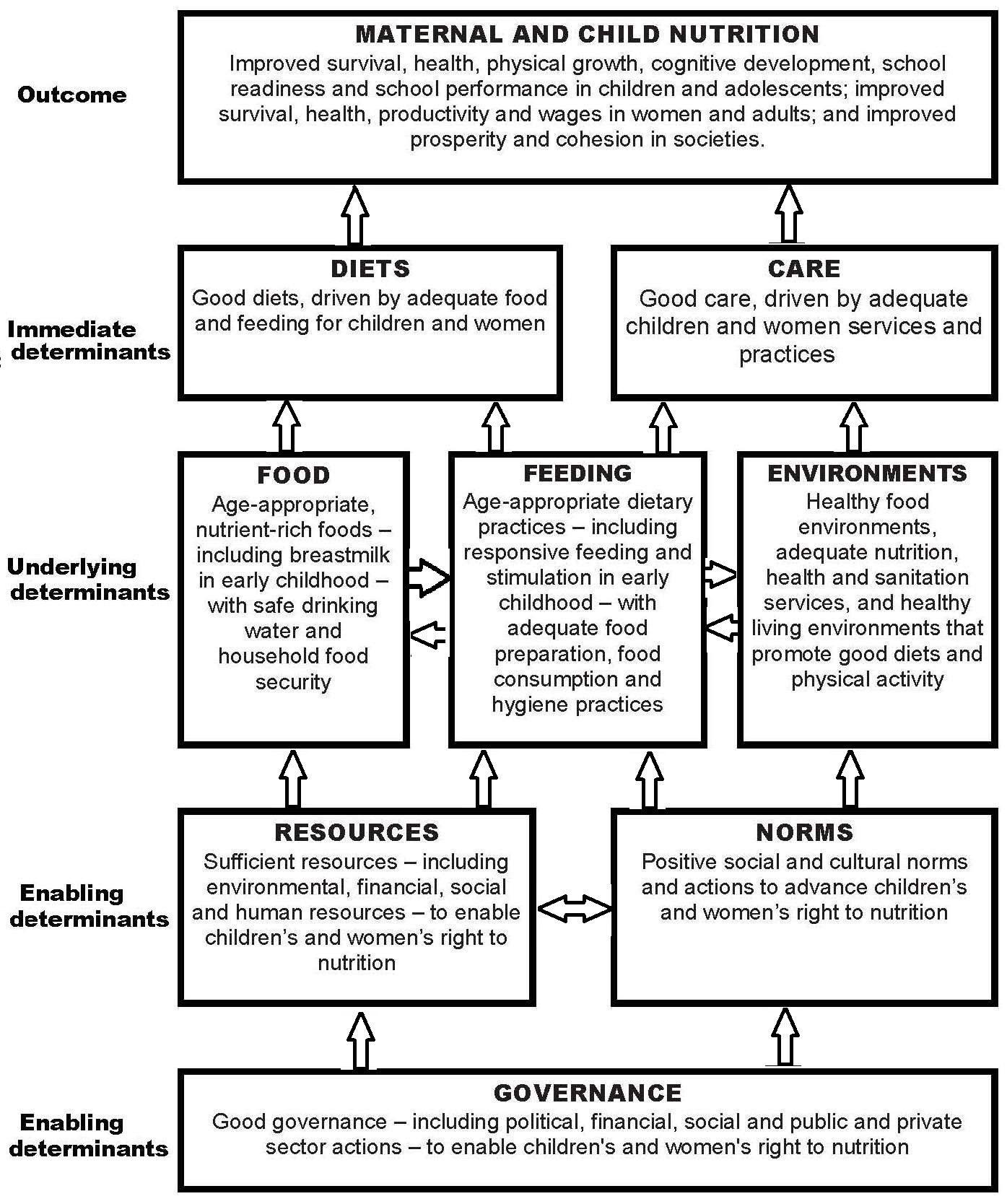
1.3 Nutritional assessment indices and indicators
Raw measurements alone have no meaning unless they are related to, for example, the age or sex of an individual (WHO, 1995). Hence, raw measurements derived from each of the four methods are often (but not always) combined to form “indices.” Examples of such combinations include height-for-age, nutrient density (nutrient intake per megajoule), BMI ((weight kg) / (height m)2), and mean cell volume ((hematocrit) / (red blood cell count)). These indices are all continuous variables. Construction of indices is a necessary step for the interpretation and grouping of measurements collected by nutritional assessment systems, as noted earlier. Indices are often evaluated in clinical and public health settings by comparison with predetermined reference limits or cutoff points (Section 1.5). Reference limits in anthropometry in low income countries are often defined by Z‑scores below −2, as noted earlier. For example, children aged 6–60mos with a height-for-age Z‑score < −2 are referred to as “stunted”. When used in this way, the index (height‑for‑age) and the associated reference limit (i.e., < −2 Z‑score) are together termed an “indicator”, a term used in nutritional assessment, often for public health or social/medical decision-making at the population level. Several anthropometric indicators have been recommended by the WHO. For example, they define “underweight” as a weight-for-age < −2 Z‑score, “stunted” as length/height-for-age < −2 Z‑score), and “wasted” as weight-for-length/height < −2 Z‑score. In children aged 0–5y, WHO uses a Z‑score above +2 for BMI‑for‑age as an indicator of “overweight”, and above +3 as an indicator of obesity (de Onis and Lobstein, 2010). Anthropometric indicators are frequently combined with dietary and micronutrient biomarker indicators for use in public health programs to identify populations at risk; some examples are presented in Table 1.3.Nutritional indicator | Application |
---|---|
Dietary indicators | |
Prevalence of the population with zinc intakes below the estimated average requirement (EAR) | Risk of zinc deficiency in a population |
Proportion of
children 6–23mos of age who receive foods from 4 or more food groups | Prevalence of minimum dietary diversity |
Anthropometric indicators | |
Proportion of children
age 6–60mos in the population with mid-upper arm circumference < 115mm | Risk of severe acute malnutrition in the population |
Percentage of children < 5y with length- or height-for-age less than −2.0 SD below the age-specific median of the reference population | Risk of zinc deficiency in the population |
Lab. indicators based on micronutrient biomarkers | |
Percentage of population with serum Zn concentrations below the age/sex/time of day-specific lower cutoff | Risk of zinc deficiency in the population |
Percentage of children
age 6–71mos in the population with a serum retinol < 0.70µmol/L | Risk of vitamin A deficiency in the population |
Median urinary iodine <20µg/L based
on > 300 casual urine samples |
Risk of severe IDD in the population |
Proportion of children (of defined age and sex) with two or more abnormal iron indices (serum ferritin, erythrocyte protoporphyrin, transferrin receptor) plus an abnormal hemoglobin |
Risk of iron deficiency anemia in the population |
Clinical indicators | |
Prevalence of goiter in school-age children ≥ 30% |
Severe risk of IDD among the children in the population |
Prevalence of maternal night blindness ≥ 5% |
Vitamin A deficiency is a severe public health problem |
1.4 The design of nutritional assessment systems
The design of the nutritional assessment system is critical if time and resources are to be used effectively. The assessment system used, the type and number of measurements selected, and the indices and indicators derived from these measurements will depend on a variety of factors. Efforts have increased dramatically in the past decade to improve the content and quality of nutritional assessment systems, especially those involving clinical trials. In 2013, guidelines were published on clinical trial protocols entitled: Standard Protocol Items: Recommendations for International Trials (SPIRIT). This has led to the compulsory preregistration of clinical trials, and often publication of the trial protocols in scientific journals. The SPIRIT checklist consists of 33 recommended items to include in a clinical trial. Chan et al. (2013) provide the rationale, a detailed description, and model example of each item. Discussions on compulsory preregistration of protocols for observational studies are in progress; see Lash and Vandenbroucke (2012). An additional suggestion to support transparency and reproducibility in clinical trials, and to distinguish data-driven analyses from pre-planned analyses is the publication of a statistical analysis plan before data have been accessed (DeMets et al., 2017; Gamble et al., 2017). Initially, recommendations for a pre-planned statistical analyses plan were compiled only for clinical trials (Gamble et al., 2017), but have since been modified for observational studies by Hiemstra et al. (2019) to include details on the adjustment for possible confounders. Tables of the recommended content of statistical analysis plans for both clinical trials and observational studies are also available in Hiemstra et al. (2019).1.4.1 Study objectives and ethical issues
The general design of the assessment system, the raw measurements, and, in turn, the indices and indicators derived from these measurements should be dictated by the study objectives. Possible objectives may include:- Determining the overall nutritional status of a population or subpopulation
- Identifying areas, populations, or subpopulations at risk of chronic malnutrition
- Characterizing the extent and nature of the malnutrition within the population or subpopulation
- Identifying the possible causes of malnutrition within the population or subpopulation
- Designing appropriate intervention programs for high-risk populations or subpopulations
- Monitoring the progress of changing nutritional, health, or socioeconomic influences, including intervention programs
- Evaluating the efficacy and effectiveness of intervention programs
- Tracking progress toward the attainment of long-range goals.
Box 1.6: Some possible guidelines for research on
human subjects
A more detailed discussion of the main ethical issues when planning
an application for research ethical approval is available in
Gelling
(2016).
Informed consent must be obtained from
the participants or their principal caregivers in
all studies. When securing informed consent,
the investigator should also:
- Risks to subjects are minimized and proportional to the anticipated benefits and knowledge.
- Data are monitored to ensure safety of subjects.
- Selection of subjects is equitable.
- Vulnerable subjects, if included, are covered by additional safeguards.
- Informed consent is obtained from the subjects.
- Confidentiality is adequately protected.
- Disclose details of the nature and procedures of the study
- Clearly state the associated potential risks and benefits
- Confirm that participation in the research is voluntary
- Confirm that participants are free to withdraw from the study at any time
- Explain how the results relating to individual participants will be kept confidential
- Describe the procedures that provide answers to any questions and further information about the study.
1.4.2 Choosing the study participants and the sampling protocol
Nutritional assessment systems often target a large population — perhaps that of a city, province, or country. That population is best referred to as the “target population”. To ensure that the chosen target population has demographic and clinical characteristics of relevance to the question of interest, a specific set of inclusion criteria should be defined. However, for practical reasons, only a limited number of individuals within the target population can actually be studied. Hence, these individuals must be chosen carefully to ensure the results can be used to infer information about the target population. This can be achieved by defining a set of exclusion criteria to eliminate individuals who it would be unethical or inappropriate to study; as few exclusion criteria as possible should be specified. The technique of selecting a sample representative of the target population and of a size adequate for achieving the primary study objectives, requires the assistance of a statistician; only a very brief review is provided here. A major factor influencing the choice of the sampling protocol is the availability of a sampling frame. Additional factors include time, resources, and logistical constraints. The sampling frame is usually a comprehensive list of all the individuals in the population from which the sample is to be chosen. In some circumstances, the sampling frame may consist of a list of districts, villages, institutions, school or households, termed “sampling units” rather than individuals per se. When a sampling frame is not available, nonprobability sampling methods must be used. Three nonprobabilty sampling methods are available: consecutive sampling, convenience sampling, and quota sampling, each of which is described briefly in Box 1.7. Note that the use of nonprobability sampling methods produces samples that may not be representative of the target population and hence may lead to systematic bias: such methods should be fully documented.
Box 1.7 Nonprobability sampling protocols
Several possible sources of bias can occur when
nonprobability sampling is used, as shown by the three
domains (nos 1–3) depicted in
Figure 1.3.
Some specific examples include the following:
- Consecutive sampling is the best of the nonprobability techniques. It is used, for example, in clinical research, when it is feasible to recruit all available patients who meet the selection criteria, over a time period that is long enough to avoid seasonal factors or other changes over time.
- Convenience sampling involves taking individuals into the study who happen to be available at the time of data collection and who consent to participate. This approach is used when the population to be sampled is relatively homogeneous
- Quota sampling involves dividing the target population into a number of different categories based on age, ownership of land, or occupations etc, and taking a certain number of consenting individuals from each category into the final sample.
- Ignoring people who do not respond to an initial approach to include them in the study — the non-response bias. People who refuse to take part may have characteristics that differ markedly from those of the respondents.
- Studying only volunteers who are often unrepresentative of most of the population.
- Sampling only those persons attending a clinic, school, or health center, and neglecting to include non-attendees.
- Collecting data at only one time of the year, which may introduce a seasonal bias.
- Selecting participants who are accessible by road introduces a “tarmac” bias. Areas accessible by road are likely to be systematically different from those that are more difficult to reach.
Box 1.8 Probability sampling protocols
Cluster sampling requires defining a random
sample of natural groupings
(clusters) of individuals in the population.
This method is used when the
population is widely spaced and it is
difficult to compile a sampling frame, and
thus sample from all its elements.
Statistical analysis must take clustering into
account because cluster sampling tends to result in
more homogeneous groups for
the variables of interest in the population.
Stratified sampling results in a
sample that is not necessary representative of
the actual population. The imbalance can
be corrected, however, by weighting,
allowing the results to be generalized to the target
population. Alternatively,
a sampling strategy, termed proportional
stratification, can be used to adjust the
sampling before selecting the sample, provided
information on the size of the sampling
units is available. This approach simplifies
the data analysis and also ensures that subjects
from larger communities have a proportionately
greater chance of being selected
than do subjects from smaller communities.
Multistage random sampling is frequently used
in national nutrition surveys. It typically
involves sampling at four stages:
at the provincial or similar
level (stage one), at the
district level (stage two),
at the level of communities in each selected district
(stage three), and at the household level in each
chosen community (stage four). A random
sample must be drawn at each stage. The
U.S. NHANES III, the U.K. Diet and Nutrition
surveys, and the New Zealand and Australian
national nutrition surveys all used a combination
of stratified and multistage random
sampling techniques to obtain a sample representative
of the civilian non-institutionalized
populations of these countries.
As can be seen, each probability sampling protocol involves a
random selection procedure to ensure that
each sampling unit (often the individual) has
an equal probability of being sampled. Random
selection can be achieved by using a
table of random numbers, a computer program
that generates random numbers, or a
lottery method; each of these procedures is
described in Varkisser et al.
(1993).
- Simple random sampling involves drawing a random sample from a listing of all the people in the target population.
- Stratified random sampling divides the target population into a number of subgroups or strata (e.g., urban and rural populations, different ethnic groups, various geographical areas, or administrative regions). A separate random sample is then drawn from each of the strata. The stratified subsamples can be weighted to draw disproportionately from subgroups that are less common in the population but of special interest.
- random sampling requires defining a number of levels of sampling, from each of which is drawn a random sample.
1.4.3 Calculating sample size
The appropriate sample size for a particular nutritional assessment project should be estimated early in the process of developing the project design so that, if necessary, modifications to the design can be made. The number of participants required will depend on the study objective, the nature and scope of the study, and the “effect size” — the magnitude of the expected change or difference sought. The estimate obtained from the sample size calculation represents the planned number of individuals with data at outcome, and not the number who should be enrolled. The investigator should always plan for dropouts and individuals with missing data. The first step in the process of estimating the sample size is restating the research hypothesis to one that proposes no difference between the groups that are being compared. This restatement is called the “null” hypothesis. Next, the “alternative” hypothesis should be stated, which, if one-sided, specifies the actual magnitude of the expected “effect size” and the direction of the difference between the predictor and outcome variable. In most circumstances, however, a two-sided alternative hypothesis is stated, in which case only the effect size is specified and not the direction. The second step in the estimation of sample size is the selection of a reasonable effect size (and variability, if necessary). As noted earlier, this is rarely known, so instead both the effect size and variability must be estimated based on prior studies in the literature, or selected on the basis of the smallest effect size that would be considered clinically meaningful. Sometimes a small pilot study is conducted to estimate the variability (s2) of the variable. When the outcome variable is the change of a continuous measurement (e.g., change in a child's length during the study), the s2 used should be the variance of this change. The third step involves setting both α and β. The probability of committing a type 1 error (rejecting the null hypothesis when it is actually true) is defined as α. Another widely used name for α is the level of significance. It is often set at 0.05, when it represents a 95% assurance that a significant result will not be achieved when it should not (i.e., the null hypothesis will not be rejected). If a one-tailed alternative hypothesis has been set, then a one-tailed α should be used; otherwise, use a two-tailed α. The probability of committing a type II error (i.e.,failing to reject the null hypothesis when it is actually false) is defined as “β”, and is often set at 0.20, indicating that the investigator is willing to accept a 20% chance of missing an association of the specified effect size if it exists. The quantity 1−β is called the power, and when set at 0.80 implies there is a 80% chance of finding an association of that size or greater when it really exists. The final step involves selecting the appropriate procedure for estimating the sample size. Two different procedures can be used depending on how the effect size is specified. Frequently, the objective is to determine the sample size to detect differences in the Proportion of individuals in two groups. For example, the proportion of male infants age 9mos who develop anemia while being treated with iron supplements (Hemoglobin < 110g/L) is to be compared to the proportion who develop anemia while taking a placebo. The procedure is two‑sided, allowing for the possibility that the placebo is more effective than the supplement! Note that the effect size is the difference in the projected proportions in the two groups and that the size of that differences critically controls the required sample size. See, Sample size calculator - two proportions. In a cohort or experimental study, the effect size is the difference between P1, the proportion of individuals expected to have the outcome in one group and P2, the proportion expected in the other group. Again, this required effect size must be specified, along with α and β to calculate the required sample size. In contrast, in a case-control study, P1 represents the proportion of cases expected to have a particular dichotomous predictor variable (i.e., the prevalence of that predictor), and P2 represents the proportion of controls who are expected to have the dichotomous predictor. For examples when the effect size is specified in terms of relative risk or odds ratio (OR), see Browner et al. in Chapter 6 in Hulley et al. (2013). Alternatively, the objective may be to calculate an appropriate sample size to detect if the mean value of a continuous variable in one group differs significantly from the mean of another group. For example, the objective might be to examine the mean HAZ‑score of city childen aged 5y with their rural counterparts aged 5y. The sample size procedure assumes that the distribution of the variable in each of the two groups will be approximately normal. However, the method is statistically robust, and can be used in most situations with more than about 40 individuals in each group. Note that in this cases the effect size is the numerical difference in the means of the two groups and that the group variance must also be defined. See, Sample size calculator - two means. However,this sample size calculator cannot be used for studies involving more than two groups, when more sophisticated procedures are needed to determine the sample size. A practical guide to calculating the sample size is published by WHO (Lwanga and Lemeshow, 1991). The WHO guide provides tables of minimum sample size for various study conditions (e.g., studies involving population proportion, odds ratio, relative risk, and incidence rate), but the tables are only valid when the sample is selected in a statistically random manner. For each situation in which sample size is to be determined, the information needed is specified and at least one illustrative example is given. In practice, the final sample size may be constrained by cost and logistical considerations.1.4.4 Collecting the data
Increasingly, digital tablets rather than paper-based forms are used for data collection. Their use reduces the risk of transcription errors, and can protect data security through encryption. The transport and storage of multiple paper forms is eliminated and costs can be reduced by the elimination of extensive data entry. Several proprietary and open-source software options (e.g., Open Data Kit) are available for data collection. Initially, data are usually collected and stored locally offline, but uploaded on to a secure central data store when internet access is available. The process of data aquisition, organisation, and storage should be carefully planned in advance, with the objective of facilitating subsequent data handling and analysis and minimising data entry errors — a particular problem with dietary data.1.4.5 Additional considerations
Of the many additional factors affecting the design of nutritional assessment systems, the acceptability of the method, respondent burden, equipment and personnel requirements, and field survey and data processing costs are particularly important. The methods should be acceptable to both the target population and the staff who are performing the measurements. For example, in some settings, drawing venous blood for biochemical determinations such as serum retinol may be unacceptable in infants and children, whereas the collection of breast milk samples may be more acceptable. Similarly, collecting blood specimens in populations with a high prevalence of HIV infections may be perceived to be an unacceptable risk by staff performing the tests. To reduce the nonresponse rate and avoid bias in the sample selection, the respondent burden should be kept to a minimum. In the U.K. Diet and Nutrition Survey, the seven-day weighed food records were replaced by a four-day estimated food diary, when the rolling program was introduced in 2008 due to concerns about respondent burden (Ashwell et al., 2006). Alternative methods for minimizing the nonresponse rate includes the offering of material rewards and the provision of incentives such as regular medical checkups, feedback information, social visits, and telephone follow-up. The requirements for equipment and personnel should also be taken into account when designing a nutritional assessment system. Measurements that require elaborate equipment and highly trained technical staff may be impractical in a field survey setting; instead, the measurements selected should be relatively noninvasive and easy to perform accurately and precisely using rugged equipment and unskilled but trained assistants. The ease with which equipment can be transported to the field, maintained, and calibrated must also be considered. The field survey and data processing costs are also important factors. Increasingly, digital tablet devices are being used for data collection in field surveys rather than paper-based forms. As noted earlier, adoption of this method reduces the risk of transcribing error, protects data security through encryption and reduces the cost of extensive data entry. Several proprietary and open-source software options are available, including Open Data Kit, RedCap, and Survey CTO. Software such as Open Data Kit permits offline data collection, automatic encryption, and the ability to upload all submissions when a data collection devise, such as a notebook, is connected to the internet. In surveillance systems, the resources available may dictate the number of malnourished individuals who can subsequently be treated in an intervention program. When resources are scarce, the cutoff point for the measurement or test (Section 1.5.3) can be lowered, a practice that simultaneously decreases sensitivity, but increases specificity, as shown in Table 1.7. As a result, more truly malnourished individuals will be missed while at the same time fewer well-nourished individuals are misdiagnosed as malnourished.1.5 Important characteristics of assessment measures
All assessment measures vary in their validity, sensitivity, specificity, and predictive value; these characteristics, as well as other important attributes, are discussed below,1.5.1 Validity
Validity is an important concept in the design of nutritional assessment systems. It describes the adequacy with which a measurement or indicator reflects what it is intended to measure. Ideally valid measures are free from random and systematic errors and are both sensitive and specific (Sections 1.5.4; 1.5.5; 1.5.7; 1.5.8). In dietary assessment, a method that provides a valid reflection of the true “usual nutrient intake” of an individual is often required. Hence, a single weighed food record, although the most accurate dietary assessment method, would not provide a valid assessment of the true “usual nutrient intake” of an individual, but instead provides a measurement of the actual intake of an individual over one day. Similarly, if the biomarker selected reflects “recent” dietary exposure, but the study objective is to assess the total body store of a nutrient, the biomarker is said to be invalid. In the earlier U.S. NHANES I survey, thiamine and riboflavin were analyzed in casual urine samples because it was not practical to collect 24h urine samples. However, the results were not indicative of body stores of thiamine or riboflavin, and hence were considered invalid; the determination of thiamine and riboflavin in casual urine samples were not included in U.S. NHANES II or U.S. NHANES III (Gunter and McQuillan, 1990). In some circumstances, assessment measures only have “internal” validity, indicating that the results are valid only for the particular group of individuals being studied and cannot be generalized to the universe. In contrast, if the results have “external” validity, or generalizability, then the results are valid when applied to individuals not only in the study but in the wider universe as shown in Figure 1.6.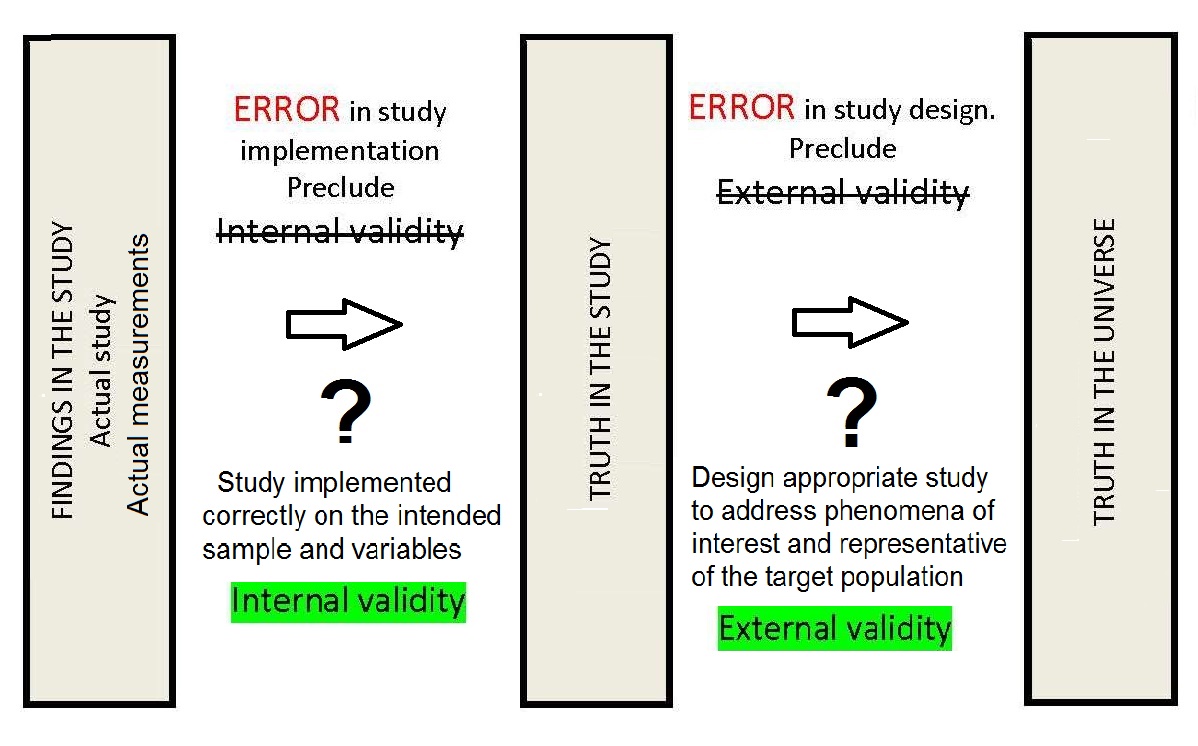
1.5.2 Reproducibility or precision
The degree to which repeated measurements of the same variable give the same value is a measure of reproducibility — also referred to as “reliability” or “precision” in anthropometric (Chapter 9) and laboratory assessment (Chapter 15). The measurements can be repeated on the same subject or sample by the same individual (within-observer reproducibility) or different individuals (between-observer reproducibility). Alternatively, the measurements can be assessed within or between instruments. Reproducible measurements yield greater statistical power at a given sample size to estimate mean values and to test hypotheses. The study design should always include some replicate observations (repeated but independent measurements on the same subject or sample). In this way, the reproducibility of each measurement can be calculated. When the measurements are continuous, the coefficient of variation (CV%) can be calculated: \[\small \mbox {CV %= standard deviation × 100% / mean}\] For categorical variables, percent agreement, the interclass correlation coefficient, and the kappa statistic can be used. In anthropometry, alternative methods are often used to assess the precision of the measurement techniques; these are itemized in Box 1.9, and discussed in Chapter 9. The TEM was calculated for each anthropometric measurement used in the WHO Multicenter Growth Reference Study for the development of the Child Growth Standards; see de Onis et al. (2004).
Box 1.9 Measures of the precision of anthropometric measurements
The reproducibility of a measurement is a
function of the random measurement errors
(Section 1.5.4)
and, in certain cases, true variability in the
measurement that occurs over time. For example,
the nutrient intakes of an individual
vary over time (within-person variation), and
this results in uncertainty in the estimation of
usual nutrient intake. This variation characterizes
the true “usual intake” of an individual.
Unfortunately, within-person variation
cannot be distinguished statistically from random
measurement errors, irrespective of the
design of the nutritional assessment system
(see Chapter 6 for more details).
The precision of biochemical measures is
similarly a function of random errors that
occur during the actual analytical process and
within-person biological variation in the biochemical
measure. The relative importance of
these two sources of uncertainty vary with
the different measures. For many modern biochemical
measures, the within-person biological
variation now exceeds the long-term analytical
variation, as shown in
Table 1.4.
- Technical Error of the Measurement (TEM)
- Percentage Technical error (% TEM)
- Coefficient of Reliability
Coefficient of variation (%) | ||
---|---|---|
Measurement | Within-person | Analytical |
Serum retinol | ||
Daily | 11.3 | 2.3 |
Weekly | 22.9 | 2.9 |
Monthly | 25.7 | 2.8 |
Serum ascorbic acid | ||
Daily | 15.4 | 0.0 |
Weekly | 29.1 | 1.9 |
Monthly | 25.8 | 5.4 |
Serum albumin | ||
Daily | 6.5 | 3.7 |
Weekly | 11.0 | 1.9 |
Monthly | 6.9 | 8.0 |
- Compiling an operations manual that contains specific written guidelines for taking each measurement, to ensure all the techniques are standardized
- Training all the examiners to use the standardized techniques consistently; the latter is especially important in large surveys involving multiple examiners, and in longitudinal studies, where maintaining standardized measurement techniques during the survey is an important issue
- Carefully selecting and standardizing the instruments used for the data collection; in some cases, variability can be reduced by the use of automated instruments
- Refining and standardizing questionnaires and interview protocols, preferably, where feasible, with the use of computer-administered interview protocols; the latter approach is now often used for 24-h recall interviews in national nutrition surveys
- Reducing the effect of random errors from any source by repeating all the measurements, when feasible, or at least on a random subsample.
1.5.3 Accuracy
The term “accuracy” is best used in a restricted statistical sense to describe the extent to which the measurement is close to the true value. It therefore follows that a measurement can be reproducible or precise, but, at the same time, inaccurate — a situation which occurs when there is a systematic bias in the measurement (see Figure 1.7 and Section 1.5.5). The greater the systematic error or bias, the less accurate the measurement. Accurate measurements, however, necessitate high reproducibility, as shown in Figure 1.7.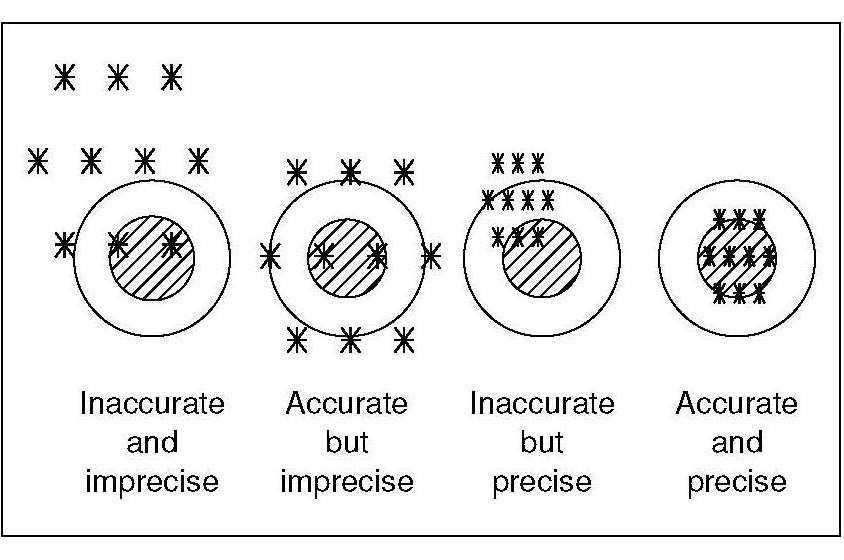
Precision or reproducibility | Accuracy | |
---|---|---|
Definition |
The degree to which repeated measurements of the same variable give the same value |
The degree to which a measurement is close to the true value |
Assess by | Comparison among repeated measures | Comparison with certified reference materials, criterion method, or criterion anthropometrist |
Value to study | Increases power to detect effects | Increases validity of conclusions |
Adversely affected by |
Random error contributed by the measurer, the respondent, or the instrument | Systematic error (bias) contributed by: the measurer, the respondent, or the instrument |
1.5.4 Random errors
Random errors generate a deviation from the correct result due to chance alone. They lead to measurements that are imprecise in an unpredictable way, resulting in less certain conclusions. They reduce the precision of a measurement by increasing the variability about the mean. They do not influence the mean or median value. There are three main sources of random error:- Individual biological variation
- Sampling error
- Measurement error
1.5.5 Systematic errors or bias
Unfortunately, systematic errors may arise in any nutritional assessment method, causing it to become biased. Bias may be defined as a condition that causes a result to depart from the true value in a consistent direction. The errors arising from bias reduce the accuracy of a measurement by altering the mean or median value. They have no effect on the variance and hence do not alter the reproducibility or precision of the measurement (Himes, 1987). Several types of bias exist, as shown in Figure 1.3. A detailed list of sources of bias that can affect nutrition studies is available in Yetley et al. (2017a), but the principal biases are selection bias and measurement bias. All types of nutritional assessment systems may experience selection bias. It arises when there is a systematic difference between the characteristics of the individuals selected for the study and the characteristics of those who are not, making it impossible to generalize the results to the target population. Selection bias may originate in a variety of ways. Some of these are outlined in Box 1.10.
Box 1.10: Various types of selection bias
Wherever possible, a strategy should be
used to obtain information on people who
refuse to participate or subsequently fail to
complete the study. This information can
then be used to assess whether those who did
not participate or dropped out of the study are
similar to the participants. If they differ, then
a selection bias is present.
Measurement bias can be introduced in a
variety of ways. For example:
Biased equipment may over- or underestimate
weight or height. Alternatively,
skinfold calipers may systematically over- or
under-estimate skinfold thickness because
of differences in the degree of compression
arising from the magnitude of the jaw pressure.
Analytical bias may result from the use of
a biochemical method that systematically
under- or overestimates the nutrient content
of a food or biological specimen. For example,
vitamin C may be underestimated because
only the reduced form of vitamin C,
and not total vitamin C, is measured.
Alternatively, if the biopsy specimens of the
treatment and control groups are
analyzed in different laboratories
which produce systematically different results for
the same assay, then the assay results will be biased.
Social desirability bias occurs, for example,
when respondents underestimate their
alcohol consumption in a 24h food recall or record.
However, scales can be used to measure
the extent of the bias
(Robinson et al., 1991),
including the Marlowe-Crowne Social Desirability Scale
(Crowne and Marlowe, 1960).
Interviewer bias arises when interviewers
differ in the way in which they obtain,
record, process, and interpret information.
This is a particular problem if different interviewers
are assigned different segments
of the population, such as different racial
or age groups.
Recall bias is a form of measurement bias
of critical importance in retrospective case control
studies. In such studies, there
may be differential recall of information
by cases and controls. For example, persons
with heart disease will be more likely
to recall past exposure to saturated fat than
the controls, as saturated fat is widely
known to be associated with heart disease.
Such a recall bias may exaggerate the
degree of effect of association with the exposure
or, alternatively, may underestimate
the association if the cases are more likely
than controls to deny past exposure.
Bias is important as it cannot be removed by
subsequent statistical analysis. Consequently,
care must be taken to reduce and, if possible,
eliminate all sources of bias in the nutritional
assessment system by the choice of an appropriate
design and careful attention to the
equipment and methods selected. Strategies
for controlling bias and its potential effect on
the measurement of a cause-effect relationship
are described in most standard epidemiological
texts; see Hulley et al.
(2013)
for more details.
For examples of criteria that can be applied to
assess the risk of bias depending on
the type of study (i.e., RCT, cohort,
case-control, cross-sectional), see Yetley et al.
(2017a).
- Self-selection bias results from studying only volunteers for a study, who perhaps volunteer because they are unwell.
- Referral bias will be present if cases are recruited through a district health center but controls from an adjacent village.
- nonresponse bias is caused by ignoring people who do not respond to an initial attempt to include them in the study.
- Diagnostic bias arises from selecting subjects for a multicenter case-control study using different diagnostic criteria in different centers.
- Drop-out bias is usually the result of ignoring possible systematic differences between those who fail to complete a study and the remaining participants.
1.5.6 Confounding
Confounding is a special type of bias that can affect the validity of a study: it masks the true effect. A confounding variable is defined as a characteristic or variable that is associated with the problem and with a possible cause of the problem. See Howards (2018a; and 2018b). Such a characteristic or variable may either strengthen or weaken the apparent relationship between the problem and possible cause. Three conditions must exist for confounding to occur. These are:- Confounding factor must be associated with both the risk factor of interest and the outcome;
- Confounding factor must be distributed unequally among the groups being compared;
- A confounder cannot be an intermediary step in the causal pathway from the exposure of interest to the outcome of interest.
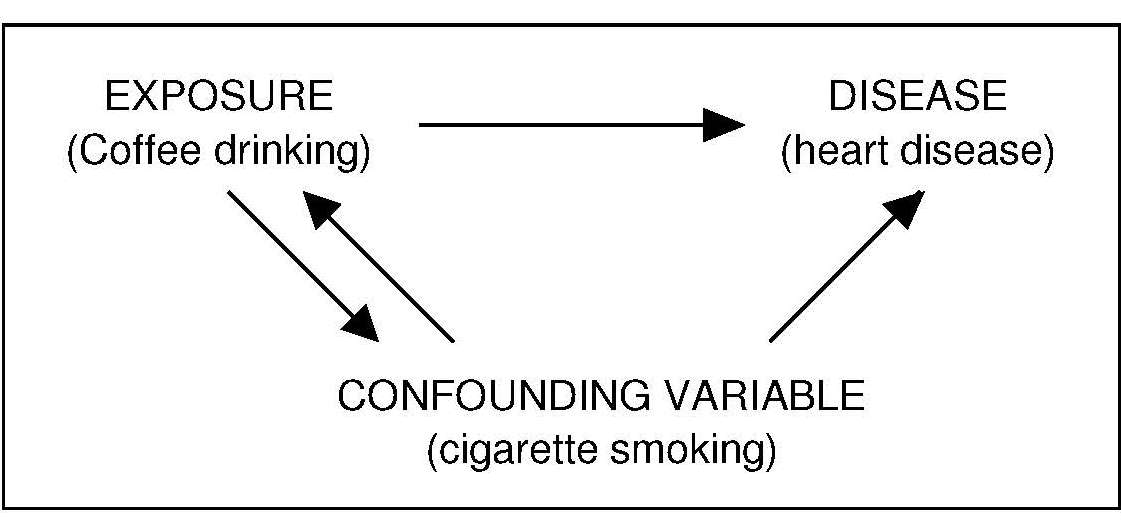
1.5.7 Sensitivity
The sensitivity of a test or indicator refers to the extent to which it reflects nutritional status or predicts changes in nutriture. Sensitive tests (or indicators) show large changes as a result of only small changes in nutritional status. As a result, they have the ability to identify and classify those persons within a population who are genuinely malnourished. Some variables are strictly homeostatically controlled, and hence have very poor sensitivity. An example is shown in Figure 1.9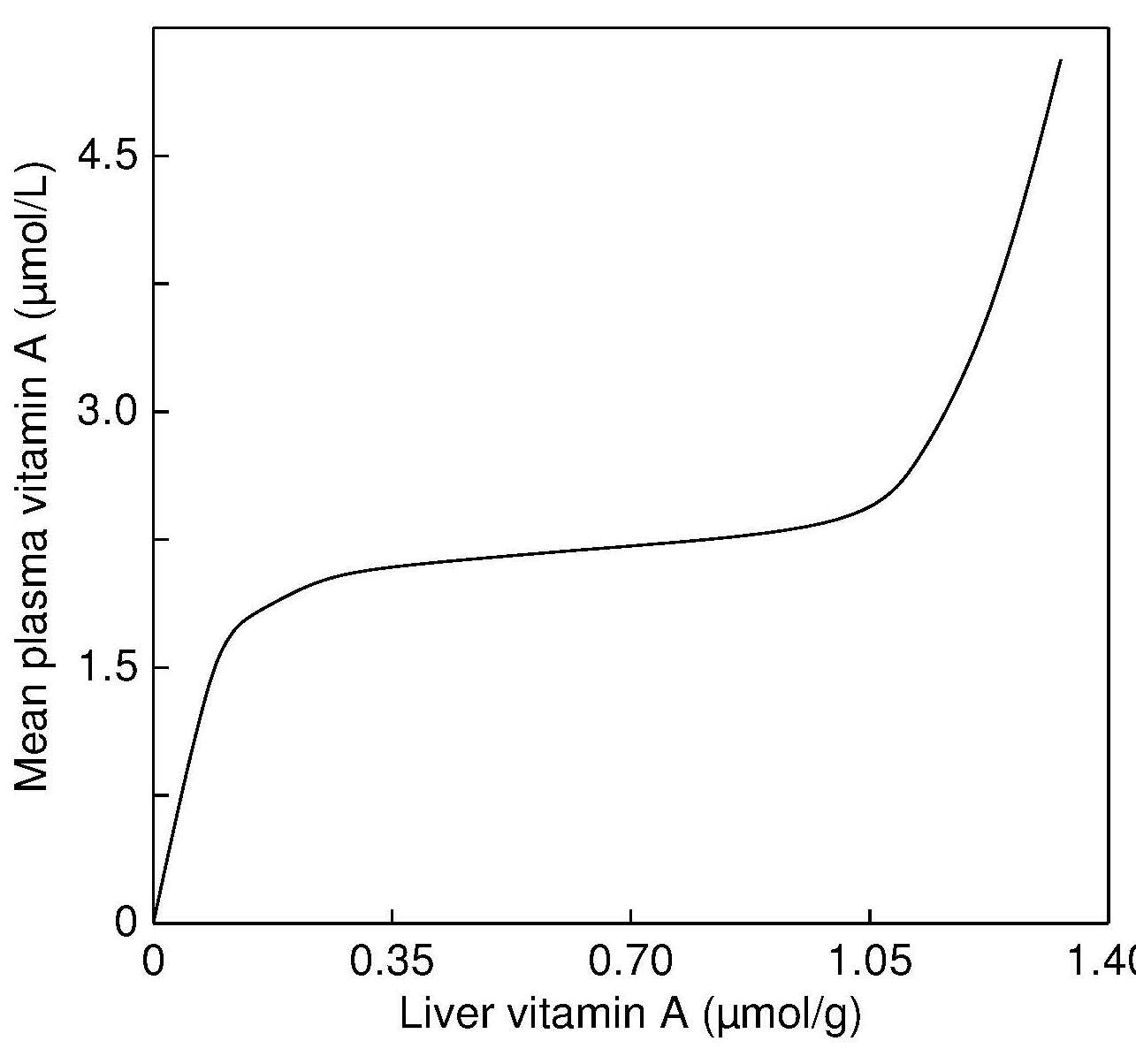
1.5.8 Specificity
The specificity of a test (or indicator) refers to the ability of the test (or indicator) to identify and classify those persons who are genuinely well nourished. If a measurement (or indicator) has 100% specificity, all genuinely well-nourished individuals will be correctly identified: no well-nourished individuals will be classified as “ill” (i.e., there are no false positives). Numerically, specificity (Sp) is the proportion of individuals without malnutrition who have negative tests (true negatives divided by the sum of true negatives and false positives). Table 1.6Test result | The true situation: Malnutrition present | The true situation: No malnutrition |
---|---|---|
Positive | True positive (TP) | False positive (FP) |
Negative | False negative (FN) | True negative (TN) |
Arm circum- ference (mm) | Sensitivity (%) | Specificity (%) |
Relative Risk of death |
---|---|---|---|
≤ 100 | 42 | 99 | 48 |
100–110 | 56 | 94 | 20 |
110–120 | 77 | 77 | 11 |
120–130 | 90 | 40 | 6 |
Biomarker in serum | Geometric mean (95% CI) | Proportion at risk (%) |
---|---|---|
Ferritin*: No adjustment | 14.5µg/L (13.6–17.5) | 44.9 |
Ferritin: Brinda adjustment | 8.8µg/L (8.0–9.8) | 64.9 |
Retinol binding protein**: No adjustment | 0.98 (µmol/L) (0.94–1.01) | 24.3 |
Retinol binding protein: Brinda adjustment | 1.07µmol/L (1.04–1.10) | 12.4 |
Zinc***: No adjustment | 11.5µmol/L (11.2–11.7) | 13.0 |
Zinc: Brinda adjustment | 11.7µmol/L (11.4–12.0) | 10.4 |
1.5.9 Prevalence
The number of persons with malnutrition or disease during a given time period is measured by the prevalence. Numerically, the actual prevalence (P) is the proportion of individuals who really are malnourished or infected with the disease in question (the sum of true positives and false negatives) divided by the sample population (the sum of true positives, false positives, true negatives, and false negatives) (Table 1.6). Prevalence influences the predictive value of a nutritional index more than any other factor (see Section 1.5.10). For example, when the prevalence of malnutrition such as anemia decreases, it becomes less likely that an individual with a positive test (i.e., low hemoglobin) actually has anemia and more likely that the test represents a false positive. Therefore, the lower the prevalence of the condition, the more specific a test must be to be clinically useful (Hulley et al., 2013).1.5.10 Predictive value
The predictive value can be defined as the likelihood that a test correctly predicts the presence or absence of malnutrition or disease. Numerically, the predictive value of a test is the proportion of all tests that are true (the sum of the true positives and true negatives divided by the total number of tests) (Table 1.6). Because it incorporates information on both the test and the population being tested, predictive value is a good measure of overall clinical usefulness. The predictive value can be further subdivided into the positive predictive value and the negative predictive value, as shown in Table 1.6. The positive predictive value of a test is the proportion of positive tests that are true (the true positives divided by the sum of the true positives and false positives). The negative predictive value of a test is the proportion of negative tests that are true (the true negatives divided by the sum of the true negatives and false negatives). In other words, positive predictive value is the probability of the person having malnutrition or a disease when the test is positive, whereas negative predictive value is the probability of the person not having malnutrition or disease when the test is negative. The predictive value of any test is not constant but depends on the sensitivity and specificity of the test, and most importantly, on the prevalence of malnutrition or disease in the population being tested. Table 1.9 shows the influence of prevalence on the positive predictive value of an index when the sensitivity and specificity are constant. When the prevalence of malnutrition is low, even very sensitive and specific tests have a relatively low positive predictive value. Conversely, when the prevalence of malnutrition is high, tests with rather low sensitivity and specificity may have a relatively high positive predictive value (Table 1.9).Predictive Value | Prevalence 0.1% 1% 10% 20% 30% 40% |
---|---|
Positive | 0.02 0.16 0.68 0.83 0.89 0.93 |
Negative | 1.00 1.00 0.99 0.99 0.98 0.97 |
1.6 Evaluation of nutritional assessment indices
In population studies, nutritional assessment indices can be evaluated by comparison with a distribution of reference values from a healthy population (if available) using percentiles, standard deviation scores (Z‑scores), and in some cases, percent-of-median (See Chapter 13 for more details). Alternatively, for classifying individuals, the values for nutritional assessment indices can be compared with either statistically predetermined reference limits drawn from the reference distribution for a healthy population or cutoff points. The latter are based on data that relate the levels of the indices to low body stores of the nutrient, impaired function, clinical signs of deficiency, morbidity or mortality. Sometimes, more than one reference limit or cutoff point is used to define degrees of malnutrition (e.g., undernutrition, overweight, obesity with body mass index) (Chapters 9 and 10).1.6.1 Reference distribution
Reference values are obtained from the reference sample group. The distribution of these reference values form the reference distribution. The relationship between the terms used to define reference values is shown in Box 1.11Box 1.11 The relationship between the reference
population, the reference distribution, and reference limits
Theoretically, only healthy persons are included
in the reference sample group. However,
few “true” healthy reference distributions
have been compiled. Exceptions include the
distributions of growth reference
values for the new WHO Child
Growth Standards for children
aged 0–60mos.
These describe the growth of
children whose care has followed recommended
health practices and behaviors
associated with healthy outcomes.
Hence, they are said to be prescriptive,
depicting physiological human growth
for
children 0–60mos under optimal
conditions; for more details
see de Onis et al.
(2004).
The distribution of reference
values for hemoglobin (by age, sex, and race) compiled
from U.S. NHANES III (1988–1991),
and for serum zinc (by age, sex, blood collection time/fasting status)
compiled from U.S. NHANES 1I (1976-1980),
are other examples of a “true” healthy
reference distributions. They are based on a sample of
healthy, nonpregnant individuals, with data
from any person with conditions known to affect
iron status, or in the second case serum zinc concentrations, excluded
(Looker et al., 1997;
Hotz et al., 2003).
In practice, however, more frequently the reference values for the
reference sample group are
drawn from the general population sampled
during a nationally representative survey such
as U.S. NHANES III
(1988–1994)
or the U.K. National Diet and
Nutrition surveys.
For comparison of the observed values at the
individual or population level
with data derived from the reference
sample, the person(s) under observation
should be matched as closely as possible
to the reference individuals by the factors
known to influence the measurement
(Ritchie and Palomaki, 2004).
Frequently, these factors include age, sex, race,
and physiological state, and, depending on
the variable, they may also include exercise,
body posture, and fasting status.
In Figure 1.10,
the distribution of length/height-for-age scores of male children
participating in the Indian National Family Health Survey (2005–2006)
are matched by age and sex with the WHO Child
Growth Standard for children 0–5y.
The time of day used for specimen collection
is especially critical for comparison of serum zinc concentrations
with reference data
(Hotz et al., 2003).
Only if these matching
criteria are met can the observed value be correctly interpreted.
Figure 1.10
- REFERENCE INDIVIDUALS
↓ make up a - REFERENCE population
↓ from which is selected a - REFERENCE SAMPLE GROUP
↓ on which are determined - REFERENCE VALUES
↓ on which is observed a - REFERENCE Distribution
↓ from which are calculated - REFERENCE LIMITS
↓ that may define - REFERENCE INTERVALS
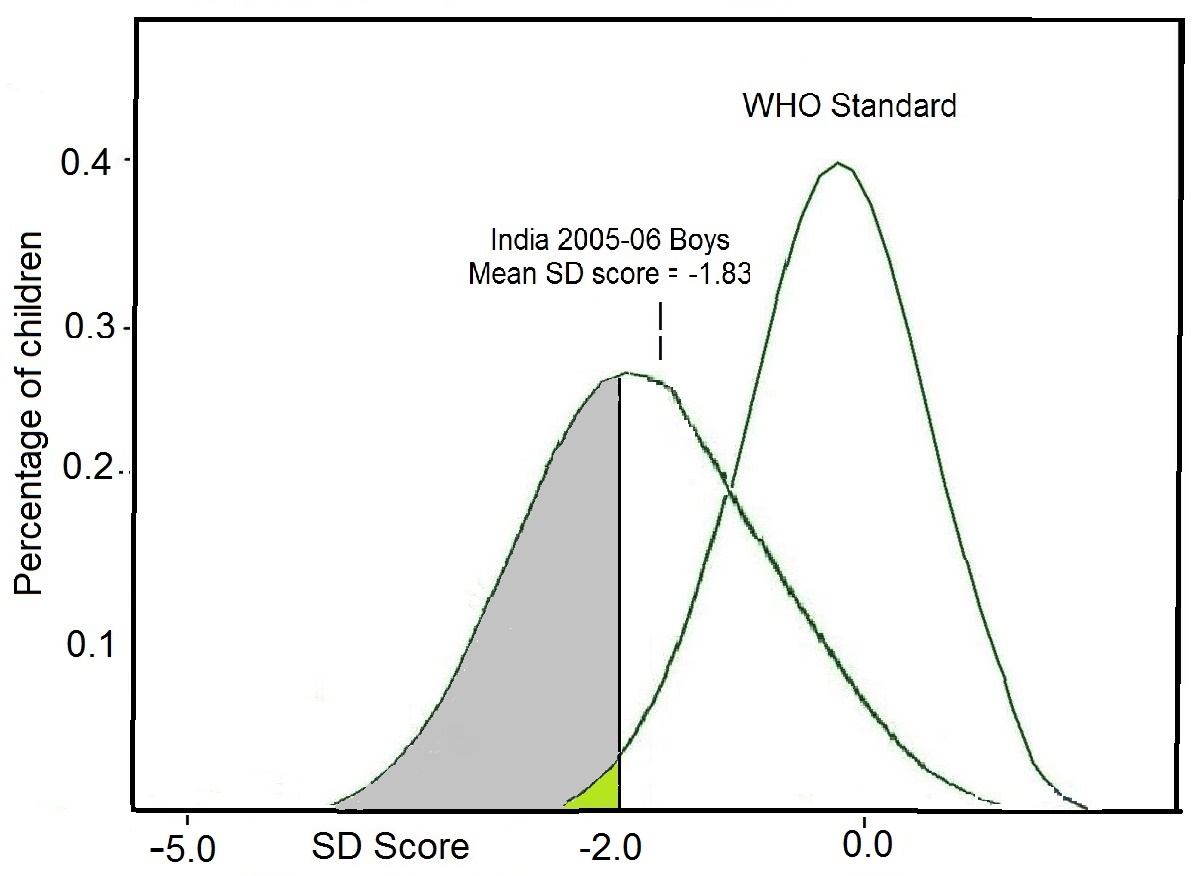
1.6.2 Reference limits
The reference distribution can also be used to derive reference limits and a reference interval. Reference limits are generally defined so that a stated fraction of the reference values would be less than or equal to the limit, with a stated probability. Two reference limits may be defined statistically, and the interval between and including them is termed the “reference interval”. Statistically, the reference interval is often the central 95% of a normal reference distribution, and is assumed to represent a normal range. Observed values for individuals can then be classified as “unusually low”, “usual”, or “unusually high,” according to whether they are situated below the lower reference limit, between or equal to either of the reference limits, or above the upper reference limit (IFCC,1984). In low income countries, reference limits for anthropometric growth indices based on Z‑scores are preferred, with Z‑scores below −2 or above +2 often used as the reference limits. to designate individuals with either unusually low or unusually high anthropometric indices. When this approach is used, theoretically the proportion of children with a Z‑score less than −2 or greater than +2 in a study population should be about 2.3%. Clearly, if the proportion in the study population with such low or high Z-scores is significantly greater than this, then the study population is seriously affected, as shown in Figure 1.10. The use of Z‑scores is recommended in low income countries because Z‑scores can be calculated accurately beyond the limits of the original reference data. In contrast, in industrialized countries, the 3rd or 5th and 95th or 97th percentiles are frequently the reference limits used to designate individuals with unusually low or unusually high anthropometric growth indices. Often for biochemical indices, only a lower reference limit is defined. In U.S. NHANES III, the lower reference limit for hemoglobin corresponded to the 5th percentile of the “true” healthy reference distribution of the U.S. NHANES III (1988–1991) survey, whereas for serum zinc, the lower reference limits (by age, sex, fasting status, and time of blood collection) are based on the 2.5th percentile values from a “true” healthy reference sample derived from U.S. NHANES II (1976–1980). See Chapter 24 and Hotz et al. (2003). Note that the terms “abnormal,” or “pathological” should not be applied when using this statistical approach for setting the reference limits because an unusually high or low value for an index is not necessarily associated with any impairment in health status (Smith et al., 1985).1.6.3 Cutoff points
Cutoff points, unlike statistically defined reference limits, are based on the relationship between nutritional assessment indices and low body stores, functional impairment, or clinical signs of deficiency or excess, as noted earlier (Raghaven et al. 2016). Their use is less frequent than that of reference limits because information relating nutritional assessment indices and functional impairment or clinical signs of deficiency or excess is often not available. Figure 1.11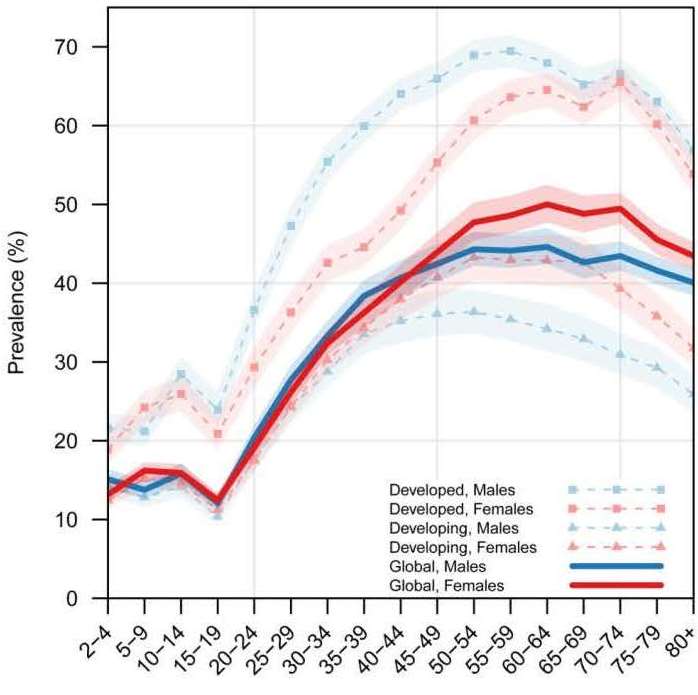
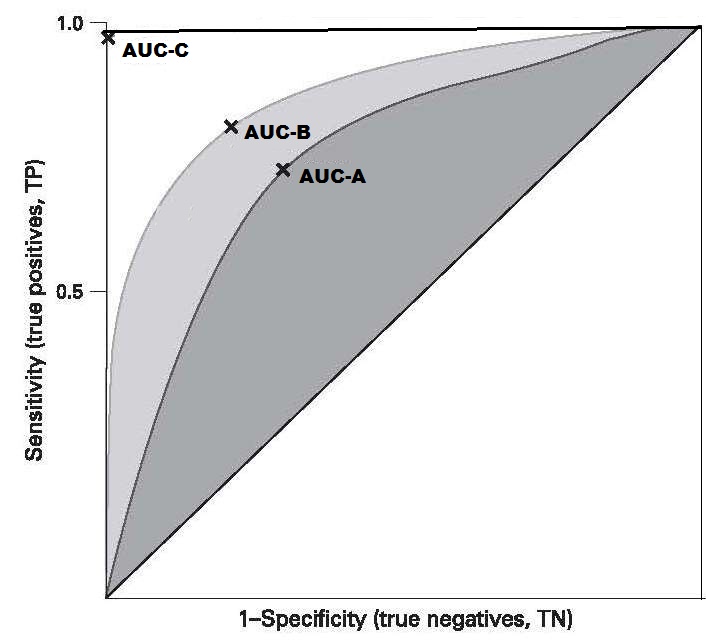
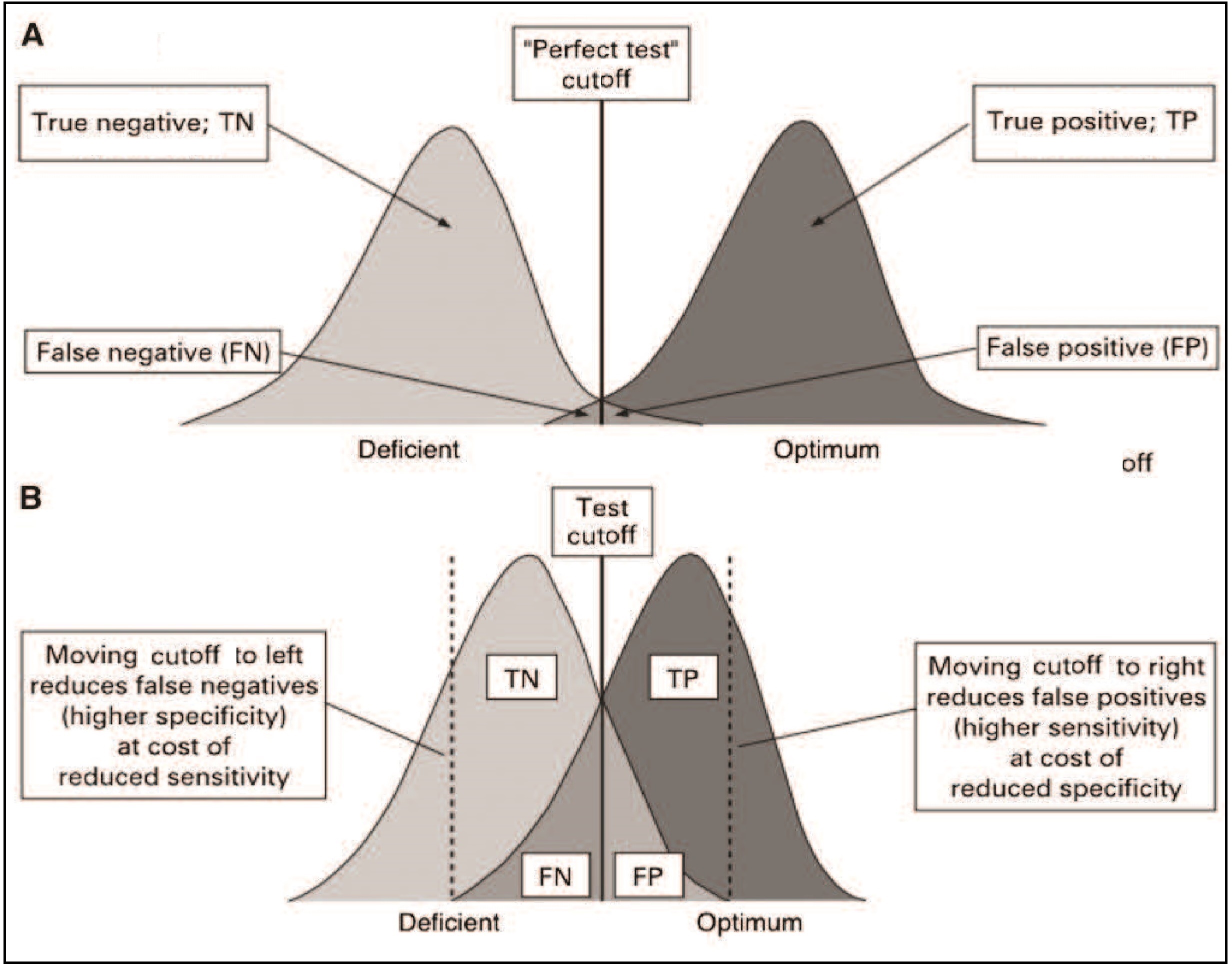
1.6.4 Trigger levels for surveillance and public health decision making
In population studies, cutoff points may be combined with trigger levels to set the level of an index (or indicator) or combination of indices at which a public health problem exists of a specified level of concern. Trigger levels may highlight regions or populations, where specific nutrient deficiencies are likely to occur, or may serve to monitor and evaluate intervention programs. They should, however, be interpreted with caution because they have not necessarily been validated in population-based surveys. Some international organizations including WHO and UNICEF (2018), the International Vitamin A Consultative Group (Sommer and Davidson, 2002), and the International Zinc Nutrition Consultative Group (IZiNCG, 2004), for example, have defined the prevalence criteria for selected indicators within a population that signify a public health problem in relation to specific nutrients and conditions.Wasting | overweight | Stunting | ||||||
---|---|---|---|---|---|---|---|---|
Prevalence thresholds (%) | Labels | (n) | Prevalence thresholds (%) | Labels | (n) | Prevalence thresholds (%) | Labels | (n) |
< 2·5 | Very low | 36 | < 2·5 | Very low | 18 | < 2·5 | Very low | 4 |
2·5 – < 5 | Low | 33 | 2·5 – < 5 | Low | 33 | 2·5 – < 10 | Low | 26 |
5 – < 10 | Medium | 39 | 5 – < 10 | Medium | 50 | 10 – < 20 | Medium | 30 |
10 – < 15 | High | 14 | 10 – < 15 | High | 18 | 20 – < 30 | High | 30 |
≥ 15 | Very high | 10 | ≥ 15 | Very high | 9 | ≥ 30 | Very high | 44 |
Box 1.12. Trigger levels
for zinc biomarkers
Comparison of the prevalence estimates for each anthropometric
indicator can trigger countries to
identify the most appropriate intervention program to
achieve “low” or “very low” prevalence threshold levels.
Trigger levels for zinc biomarkers have been set by the
International Zinc Nutrition Consultative Group. (Box 1.12)
Note that ideally, all three types of indicators
should be used together to obtain the
best estimate of the risk of zinc
deficiency in a population and to identify specific
sub-groups with elevated risk
(de Benoist et al., 2007).
A generalized discussion of the specific
procedures used for the evaluation of dietary,
anthropometric, laboratory, and clinical
methods of nutritional assessment are discussed
more fully in Chapters 8, 13, 15, and 25, respectively.
CITE AS:
Gibson R.S., Principles of Nutritional Assessment: Introduction https://nutritionalassessment.org/- Prevalence of serum zinc less than age/sex/time-of-day specific cutoffs is > 20%
- Prevalence of inadequate zinc intakes below the appropriate Estimated Average Requirement (EAR) is > 25%
- Prevalence of low height-for-age or length-for-age Z‑scores (i.e., < −2SD) is at least 20%.
Email: Rosalind.Gibson@Otago.AC.NZ
Licensed under CC-BY-4.0
( PDF ).